AI-Powered ECG Model Shows Promise in Heart Failure Risk Prediction: JAMA
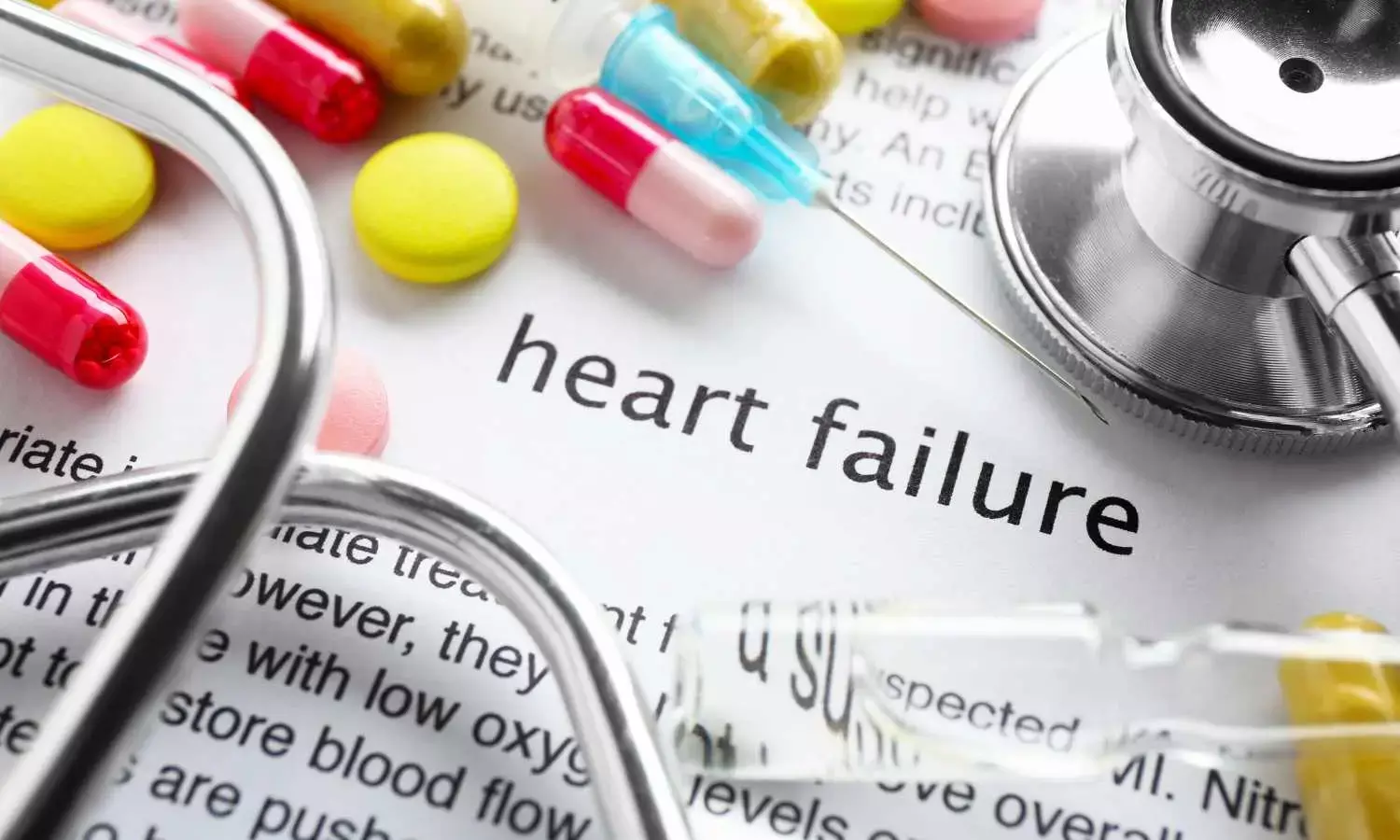
A new study published in the Journal of American Medical Association showed that a noise-adapted AI-ECG model successfully estimated heart failure risk using only lead I ECGs across diverse multinational cohorts. This suggests a potential strategy for heart failure (HF) risk stratification that could be applied using wearable and portable ECG devices, warranting further prospective studies.
Single-lead electrocardiograms (ECGs) may be recorded using portable equipment, which might allow for extensive community-based risk assessment. Thus, to determine if an artificial intelligence (AI) system can predict heart failure risk from noisy single-lead electrocardiograms, Lovedeep Dhingra and colleagues carried out this investigation.
A retrospective cohort analysis assessed persons without heart failure at baseline from UK Biobank, YNHHS, and ELSA-Brasil, utilizing outpatient ECG data. The data were evaluated between September 2023 and February 2025, with the major exposure being the AI-ECG-predicted risk of left ventricular systolic dysfunction (LVSD).
Lead I ECGs were separated to mimic wearable device signals, and a noise-adaptive AI-ECG model trained to identify LVSD was used. The model's relationship with new-onset heart failure (first HF hospitalization) was investigated. Its predictive ability was compared to the PREVENT and PCP-HF risk scores using the integrated discrimination improvement, Harrell C statistic, and net reclassification improvement.
Disclaimer: This website is primarily for healthcare professionals. The content here does not replace medical advice and should not be used as medical, diagnostic, endorsement, treatment, or prescription advice. Medical science evolves rapidly, and we strive to keep our information current. If you find any discrepancies, please contact us at corrections@medicaldialogues.in. Read our Correction Policy here. Nothing here should be used as a substitute for medical advice, diagnosis, or treatment. We do not endorse any healthcare advice that contradicts a physician's guidance. Use of this site is subject to our Terms of Use, Privacy Policy, and Advertisement Policy. For more details, read our Full Disclaimer here.
NOTE: Join us in combating medical misinformation. If you encounter a questionable health, medical, or medical education claim, email us at factcheck@medicaldialogues.in for evaluation.