- Home
- Medical news & Guidelines
- Anesthesiology
- Cardiology and CTVS
- Critical Care
- Dentistry
- Dermatology
- Diabetes and Endocrinology
- ENT
- Gastroenterology
- Medicine
- Nephrology
- Neurology
- Obstretics-Gynaecology
- Oncology
- Ophthalmology
- Orthopaedics
- Pediatrics-Neonatology
- Psychiatry
- Pulmonology
- Radiology
- Surgery
- Urology
- Laboratory Medicine
- Diet
- Nursing
- Paramedical
- Physiotherapy
- Health news
- Fact Check
- Bone Health Fact Check
- Brain Health Fact Check
- Cancer Related Fact Check
- Child Care Fact Check
- Dental and oral health fact check
- Diabetes and metabolic health fact check
- Diet and Nutrition Fact Check
- Eye and ENT Care Fact Check
- Fitness fact check
- Gut health fact check
- Heart health fact check
- Kidney health fact check
- Medical education fact check
- Men's health fact check
- Respiratory fact check
- Skin and hair care fact check
- Vaccine and Immunization fact check
- Women's health fact check
- AYUSH
- State News
- Andaman and Nicobar Islands
- Andhra Pradesh
- Arunachal Pradesh
- Assam
- Bihar
- Chandigarh
- Chattisgarh
- Dadra and Nagar Haveli
- Daman and Diu
- Delhi
- Goa
- Gujarat
- Haryana
- Himachal Pradesh
- Jammu & Kashmir
- Jharkhand
- Karnataka
- Kerala
- Ladakh
- Lakshadweep
- Madhya Pradesh
- Maharashtra
- Manipur
- Meghalaya
- Mizoram
- Nagaland
- Odisha
- Puducherry
- Punjab
- Rajasthan
- Sikkim
- Tamil Nadu
- Telangana
- Tripura
- Uttar Pradesh
- Uttrakhand
- West Bengal
- Medical Education
- Industry
Deep learning applied to ECGs could help identify patients at high risk of AF
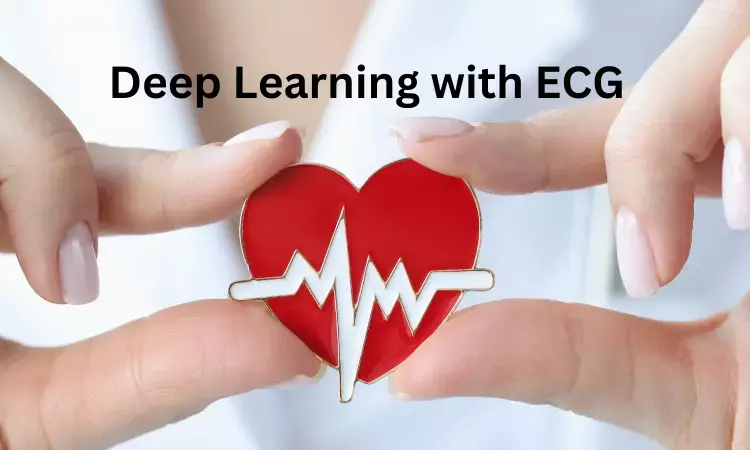
Atrial fibrillation (AF) is recognized as an irregular and often very rapid heart rhythm, affecting one-quarter of patients older than 80 years. As per studies patients with AF are 5 times more likely to experience a stroke and have up to a 25% risk of dying within 30 days of stroke. Deep learning applied to electrocardiograms (ECGs) has been successfully used for early identification of several cardiovascular diseases.
A new study in JAMA Cardiology finds that deep learning applied to ECGs could help identify patients at high risk of AF who could be considered for intensive monitoring programs to help prevent adverse cardiac events. Many cases of AF go undetected, since at least one-third are asymptomatic deep learning in ECG might be new diagnostic technique.
Researchers conducted a prognostic study was performed on ECGs acquired from January 1, 1987, to December 31, 2022, at 6 US Veterans Affairs (VA) hospital networks and 1 large non-VA academic medical center. Participants included all outpatients with 12-lead ECGs in sinus rhythm. A convolutional neural network using 12-lead ECGs from 2 US VA hospital networks was trained to predict the presence of AF within 31 days of sinus rhythm ECGs. The model was tested on ECGs held out from training at the 2 VA networks as well as 4 additional VA networks and 1 large non-VA academic medical center.
The key findings of the study are
• A total of 907 858 ECGs from patients across 6 VA sites were included in the analysis. 6.4% were female, and 93.6% were male, with CHA2DS2-VASc (congestive heart failure, hypertension, age, diabetes mellitus, prior stroke or transient ischemic attack or thromboembolism, vascular disease, age, sex category) .
• A total of 0.2% were American Indian or Alaska Native, 2.7% were Asian, 10.7% were Black, 4.6% were Latinx, 0.7% were Native Hawaiian or Other Pacific Islander, 62.4% were White, 0.4% were of other race or ethnicity (which is not broken down into subcategories in the VA data set), and 18.4% were of unknown race or ethnicity.
• At the non-VA academic medical center (72 483 ECGs), 52.5% were female, with CHA2DS2-VASc score of 1.6 (1.4).
• A total of 0.1% were American Indian or Alaska Native, 7.9% were Asian, 9.4% were Black, 2.9% were Latinx, 0.03% were Native Hawaiian or Other Pacific Islander, 74.8% were White, 0.1% were of other race or ethnicity, and 4.7% were of unknown race or ethnicity.
• A deep learning model predicted the presence of AF within 31 days of a sinus rhythm ECG on held-out test ECGs at VA sites with an area under the receiver operating characteristic curve (AUROC) of 0.86 , accuracy of 0.78, and F1 score of 0.30.
• At the non-VA site, AUROC was 0.93; accuracy, 0.87; and F1 score, 0.46. The model was well calibrated, with a Brier score of 0.02 across all sites.
• Among individuals deemed high risk by deep learning, the number needed to screen to detect a positive case of AF was 2.47 individuals for a testing sensitivity of 25% and 11.48 for 75%.
Researchers concluded that “Deep learning of outpatient sinus rhythm ECGs predicted AF within 31 days in populations with diverse demographics and comorbidities. Similar models could be used in future AF screening efforts to reduce adverse complications associated with this disease.”
Reference: Yuan N, Duffy G, Dhruva SS, et al. Deep Learning of Electrocardiograms in Sinus Rhythm From US Veterans to Predict Atrial Fibrillation. JAMA Cardiol. Published online October 18, 2023. doi:10.1001/jamacardio.2023.3701
MSc. Neuroscience
Niveditha Subramani a MSc. Neuroscience (Faculty of Medicine) graduate from University of Madras, Chennai. Ambitious in Neuro research having worked in motor diseases and neuron apoptosis is interested in more of new upcoming research and their advancement in field of medicine. She has an engrossed skill towards writing and her roles at Medical dialogue include Sr. Content writer. Her news covers new discoveries and updates in field of medicine. She can be reached at editorial@medicaldialogues.in
Dr Kamal Kant Kohli-MBBS, DTCD- a chest specialist with more than 30 years of practice and a flair for writing clinical articles, Dr Kamal Kant Kohli joined Medical Dialogues as a Chief Editor of Medical News. Besides writing articles, as an editor, he proofreads and verifies all the medical content published on Medical Dialogues including those coming from journals, studies,medical conferences,guidelines etc. Email: drkohli@medicaldialogues.in. Contact no. 011-43720751