- Home
- Medical news & Guidelines
- Anesthesiology
- Cardiology and CTVS
- Critical Care
- Dentistry
- Dermatology
- Diabetes and Endocrinology
- ENT
- Gastroenterology
- Medicine
- Nephrology
- Neurology
- Obstretics-Gynaecology
- Oncology
- Ophthalmology
- Orthopaedics
- Pediatrics-Neonatology
- Psychiatry
- Pulmonology
- Radiology
- Surgery
- Urology
- Laboratory Medicine
- Diet
- Nursing
- Paramedical
- Physiotherapy
- Health news
- Fact Check
- Bone Health Fact Check
- Brain Health Fact Check
- Cancer Related Fact Check
- Child Care Fact Check
- Dental and oral health fact check
- Diabetes and metabolic health fact check
- Diet and Nutrition Fact Check
- Eye and ENT Care Fact Check
- Fitness fact check
- Gut health fact check
- Heart health fact check
- Kidney health fact check
- Medical education fact check
- Men's health fact check
- Respiratory fact check
- Skin and hair care fact check
- Vaccine and Immunization fact check
- Women's health fact check
- AYUSH
- State News
- Andaman and Nicobar Islands
- Andhra Pradesh
- Arunachal Pradesh
- Assam
- Bihar
- Chandigarh
- Chattisgarh
- Dadra and Nagar Haveli
- Daman and Diu
- Delhi
- Goa
- Gujarat
- Haryana
- Himachal Pradesh
- Jammu & Kashmir
- Jharkhand
- Karnataka
- Kerala
- Ladakh
- Lakshadweep
- Madhya Pradesh
- Maharashtra
- Manipur
- Meghalaya
- Mizoram
- Nagaland
- Odisha
- Puducherry
- Punjab
- Rajasthan
- Sikkim
- Tamil Nadu
- Telangana
- Tripura
- Uttar Pradesh
- Uttrakhand
- West Bengal
- Medical Education
- Industry
Deep learning model may help detect type 1 MI and revascularization need from ECG patterns, reveals research
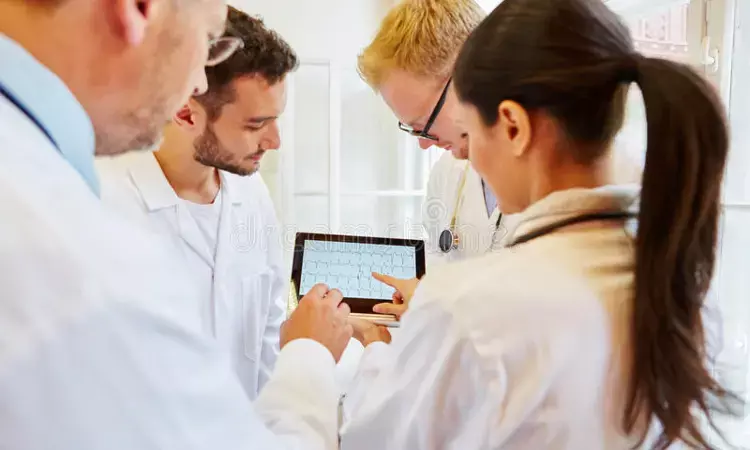
A new study published in the European Heart Journal showed that IL-Using electrocardiogram (ECG) data, a new artificial intelligence (AI)-powered tool may identify type 1 myocardial infarction (MI) more accurately at a level comparable to that of a high-sensitivity troponin T (hs-TnT) assay.
Inconclusive electrocardiogram (ECG) or biomarker data can make it difficult to identify individuals with acute coronary syndrome who need coronary revascularization. Antonius Büscher and colleagues created a deep learning model to identify ECG patterns linked to the risk of revascularization in order to direct additional evaluation and lower diagnostic ambiguity.
A convolutional neural network model was evaluated using a different test cohort (n=35,995), trained on 1,44,691 ED visits from a US cohort (60±19 years; 53% female; 0.6% revascularization), and compared to cardiac troponin T (TnT) and clinician ECG interpretation.
The results of 18,673 hospitalizations from Europe (55±21 years; 49% female; 1.5% revascularization; 1% type 1 MI) were externally validated for revascularization and type 1 MI. Area under the receiver operating characteristic curve (AUROC) served as the main performance indicator.
The model outperformed both traditional cardiac TnT (AUROC 0.71) and physician ECG interpretation in the test group, achieving an AUROC of 0.91. ECG model AUROC was 0.81 for revascularization and 0.85 for type 1 MI in the external validation cohort, while it was 0.67 and 0.74 for clinician interpretation and 0.85 and 0.87 for high-sensitivity (hs)-TnT, respectively. When compared to hs-TnT, the ECG model showed a lower sensitivity but a greater specificity.
The algorithm performed better than traditional hs-TnT testing and physicians' interpretation of the ECG in determining which individuals in the test population needed revascularization. Similar outcomes were obtained in the external validation cohort, where the ECG-AI model outperformed doctors in terms of interpretation but fell short of hs-TnT's predictive capabilities.
Overall, nearly 2,00,000 ECGs from two worldwide cohorts of patients with NSTE ACS who presented to the emergency department (ED) were used to train the deep-learning model. The findings imply that doctors may eventually get AI assistance when transferring suitable patients from the ED to the cath lab.
Reference:
Büscher, A., Plagwitz, L., Yildirim, K., Brix, T. J., Neuhaus, P., Bickmann, L., Menke, A. F., van Almsick, V. F., Pavenstädt, H., Kümpers, P., Heider, D., Varghese, J., & Eckardt, L. (2025). Deep learning electrocardiogram model for risk stratification of coronary revascularization need in the emergency department. European Heart Journal. https://doi.org/10.1093/eurheartj/ehaf254
Neuroscience Masters graduate
Jacinthlyn Sylvia, a Neuroscience Master's graduate from Chennai has worked extensively in deciphering the neurobiology of cognition and motor control in aging. She also has spread-out exposure to Neurosurgery from her Bachelor’s. She is currently involved in active Neuro-Oncology research. She is an upcoming neuroscientist with a fiery passion for writing. Her news cover at Medical Dialogues feature recent discoveries and updates from the healthcare and biomedical research fields. She can be reached at editorial@medicaldialogues.in
Dr Kamal Kant Kohli-MBBS, DTCD- a chest specialist with more than 30 years of practice and a flair for writing clinical articles, Dr Kamal Kant Kohli joined Medical Dialogues as a Chief Editor of Medical News. Besides writing articles, as an editor, he proofreads and verifies all the medical content published on Medical Dialogues including those coming from journals, studies,medical conferences,guidelines etc. Email: drkohli@medicaldialogues.in. Contact no. 011-43720751