- Home
- Medical news & Guidelines
- Anesthesiology
- Cardiology and CTVS
- Critical Care
- Dentistry
- Dermatology
- Diabetes and Endocrinology
- ENT
- Gastroenterology
- Medicine
- Nephrology
- Neurology
- Obstretics-Gynaecology
- Oncology
- Ophthalmology
- Orthopaedics
- Pediatrics-Neonatology
- Psychiatry
- Pulmonology
- Radiology
- Surgery
- Urology
- Laboratory Medicine
- Diet
- Nursing
- Paramedical
- Physiotherapy
- Health news
- Fact Check
- Bone Health Fact Check
- Brain Health Fact Check
- Cancer Related Fact Check
- Child Care Fact Check
- Dental and oral health fact check
- Diabetes and metabolic health fact check
- Diet and Nutrition Fact Check
- Eye and ENT Care Fact Check
- Fitness fact check
- Gut health fact check
- Heart health fact check
- Kidney health fact check
- Medical education fact check
- Men's health fact check
- Respiratory fact check
- Skin and hair care fact check
- Vaccine and Immunization fact check
- Women's health fact check
- AYUSH
- State News
- Andaman and Nicobar Islands
- Andhra Pradesh
- Arunachal Pradesh
- Assam
- Bihar
- Chandigarh
- Chattisgarh
- Dadra and Nagar Haveli
- Daman and Diu
- Delhi
- Goa
- Gujarat
- Haryana
- Himachal Pradesh
- Jammu & Kashmir
- Jharkhand
- Karnataka
- Kerala
- Ladakh
- Lakshadweep
- Madhya Pradesh
- Maharashtra
- Manipur
- Meghalaya
- Mizoram
- Nagaland
- Odisha
- Puducherry
- Punjab
- Rajasthan
- Sikkim
- Tamil Nadu
- Telangana
- Tripura
- Uttar Pradesh
- Uttrakhand
- West Bengal
- Medical Education
- Industry
New ML model better predicts stent restenosis risk in MI patients
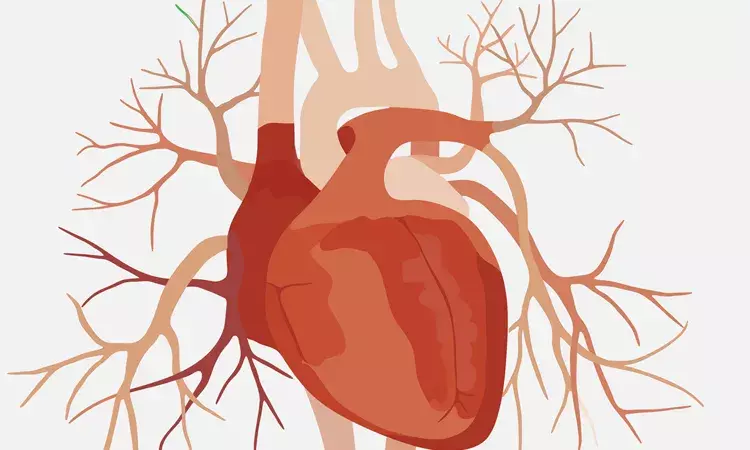
Credit: Getty Images
Spain: A new machine learning model versus currently used parameters provides a more accurate estimate of the risk for in-stent restenosis (ISR) immediately after stent implantation in patients with ST-segment elevation myocardial infarction (STEMI), according to a recent study. The study is published in the Canadian Journal of Cardiology.
According to the authors, when applied immediately after stent implantation, a new open-source machine learning (ML) model versus current discriminators better differentiates those patients who will present with SR.
Machine learning has arrived in medicine to deliver individually adapted medical care. This study by Jesús Sampedro-Gómez, Universidad de Salamanca, Salamanca, Spain, and colleagues sought to use ML to discriminate SR compared with existing predictive scores of SR. They performed predictions without any additional variables other than those obtained in daily practice in order to develop an easily applicable model.
The researchers used the dataset from GRACIA)-3 trial. It consisted of 263 patients with demographic, clinical, and angiographic characteristics; 23 (9%) of them presented with SR at 12 months after stent implantation. A methodology to work with small imbalanced datasets, based in cross-validation and the precision/recall (PR) plots, was used, and state-of-the-art ML classifiers were trained.
Key findings of the study include:
- The best performing model (0.46, area under the PR curve [AUC-PR]) was developed with an extremely randomized trees classifier, which showed better performance than chance alone (0.09 AUC-PR, corresponding to the 9% of patients presenting SR in our dataset) and 3 existing scores; Prevention of Restenosis With Tranilast and its Outcomes (PRESTO)-1 (0.31 AUC-PR), PRESTO-2 (0.27 AUC-PR), and Evaluation of Drug-Eluting Stents and Ischemic Events (EVENT) (0.18 AUC-PR).
- The most important variables ranked according to their contribution to the predictions were diabetes, ≥2 vessel-coronary diseases, post-percutaneous coronary intervention thrombolysis in myocardial infarction (PCI TIMI)-flow, abnormal platelets, post-PCI thrombus, and abnormal cholesterol.
- To counteract the lack of external validation for the study, the researchers deployed ML algorithm in an open-source calculator, in which the model would stratify patients of high and low risk as an example tool to determine the generalizability of prediction models from a small imbalanced sample size.
"Applied immediately after stent implantation, a ML model better differentiates those patients who will present with SR over current discriminators," concluded the authors.
The study, "Machine Learning to Predict Stent Restenosis Based on Daily Demographic, Clinical, and Angiographic Characteristics," is published in the Canadian Journal of Cardiology.
DOI: https://doi.org/10.1016/j.cjca.2020.01.027
Dr Kamal Kant Kohli-MBBS, DTCD- a chest specialist with more than 30 years of practice and a flair for writing clinical articles, Dr Kamal Kant Kohli joined Medical Dialogues as a Chief Editor of Medical News. Besides writing articles, as an editor, he proofreads and verifies all the medical content published on Medical Dialogues including those coming from journals, studies,medical conferences,guidelines etc. Email: drkohli@medicaldialogues.in. Contact no. 011-43720751