- Home
- Medical news & Guidelines
- Anesthesiology
- Cardiology and CTVS
- Critical Care
- Dentistry
- Dermatology
- Diabetes and Endocrinology
- ENT
- Gastroenterology
- Medicine
- Nephrology
- Neurology
- Obstretics-Gynaecology
- Oncology
- Ophthalmology
- Orthopaedics
- Pediatrics-Neonatology
- Psychiatry
- Pulmonology
- Radiology
- Surgery
- Urology
- Laboratory Medicine
- Diet
- Nursing
- Paramedical
- Physiotherapy
- Health news
- Fact Check
- Bone Health Fact Check
- Brain Health Fact Check
- Cancer Related Fact Check
- Child Care Fact Check
- Dental and oral health fact check
- Diabetes and metabolic health fact check
- Diet and Nutrition Fact Check
- Eye and ENT Care Fact Check
- Fitness fact check
- Gut health fact check
- Heart health fact check
- Kidney health fact check
- Medical education fact check
- Men's health fact check
- Respiratory fact check
- Skin and hair care fact check
- Vaccine and Immunization fact check
- Women's health fact check
- AYUSH
- State News
- Andaman and Nicobar Islands
- Andhra Pradesh
- Arunachal Pradesh
- Assam
- Bihar
- Chandigarh
- Chattisgarh
- Dadra and Nagar Haveli
- Daman and Diu
- Delhi
- Goa
- Gujarat
- Haryana
- Himachal Pradesh
- Jammu & Kashmir
- Jharkhand
- Karnataka
- Kerala
- Ladakh
- Lakshadweep
- Madhya Pradesh
- Maharashtra
- Manipur
- Meghalaya
- Mizoram
- Nagaland
- Odisha
- Puducherry
- Punjab
- Rajasthan
- Sikkim
- Tamil Nadu
- Telangana
- Tripura
- Uttar Pradesh
- Uttrakhand
- West Bengal
- Medical Education
- Industry
AI Model Predicts Atrial Fibrillation in Stroke Patients Using MRI, suggests study
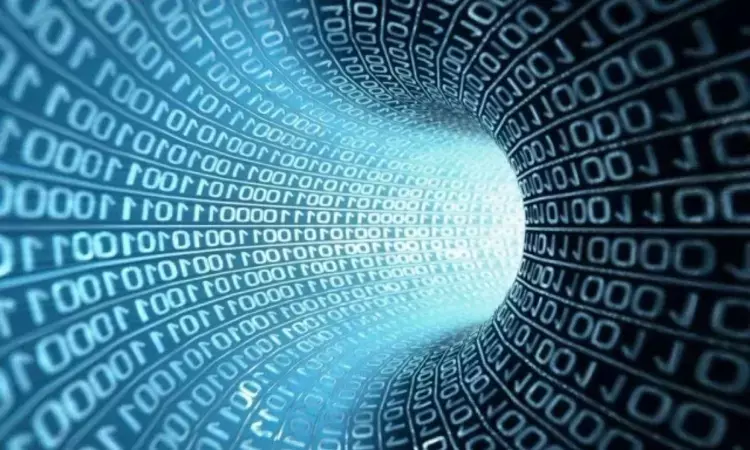
A new study published in The Lancet eClinicalMedicine journal identified an AI-driven model that can successfully forecast undiagnosed atrial fibrillation (AF) in patients presenting with acute ischemic stroke based on MRI information. AF is a key risk factor for ischemic stroke but tends to go undetected because its monitoring is not done for long durations. The study was conducted by Zijie Z. and colleagues.
A retrospective and prospective patient cohort study trained and validated an MRI-based AI model intended to predict underlying AF. The study included patients from several hospitals and evaluated the accuracy of the model with several performance measures, such as area under the curve (AUC), sensitivity, specificity, and predictive values.
The research had both retrospective and prospective patient groups from Shanghai Sixth People's Hospital, in addition to an external validation group from Huashan Hospital, Minhang Hospital, and Shanghai Tenth People's Hospital. The AI model was trained and tested on MRI data, using both pre-defined radiomics features and de novo features derived by a convolutional neural network (CNN).
• Internal retrospective cohort: 510 patients with ischemic stroke (January 1, 2018 – December 31, 2021) were retrospectively recruited for developing the algorithm. The model was tested with fivefold cross-validation.
• Prospective cohort: 73 patients (September 1, 2022 – July 31, 2023) were included to validate the real-world performance of the AI model.
• External validation cohort: 175 patients were retrospectively recruited from three hospitals to externally validate the performance of the model.
• Performance assessment measures: Area under the curve (AUC), sensitivity, specificity, accuracy, positive predictive value (PPV), and negative predictive value (NPV) were determined to evaluate the predictive power of the model.
Key Results
The AI MRI model exhibited high accuracy in the identification of AF in ischemic stroke patients across various validation groups. The joint classifier that combined radiomics and CNN-derived features resulted in the following:
• Internal retrospective validation group: AUC = 0.94 (95% CI, 0.90–0.98)
• External validation group: AUC = 0.85 (95% CI, 0.79–0.91)
• Prospective test group: AUC = 0.87 (95% CI, 0.90–0.98)
Subgroup Analysis: The AI model performed especially well in certain patient groups:
• Female patients: AUC = 0.91
• Patients with NIH Stroke Scale (NIHSS) > 4: AUC = 0.94
• Patients with CHA2DS2-VASc score ≤ 3: AUC = 0.90
The study authors concluded that MRI-Based AI-based Model is poised to be a practical screening tool for undiagnosed AF in ischemic stroke patients with catastrophic consequences. Early identification of these high-risk individuals would thus be pivotal in preventing recurrent strokes and aiding efficient management of the stroke.
Reference:
Dr Riya Dave has completed dentistry from Gujarat University in 2022. She is a dentist and accomplished medical and scientific writer known for her commitment to bridging the gap between clinical expertise and accessible healthcare information. She has been actively involved in writing blogs related to health and wellness.
Dr Kamal Kant Kohli-MBBS, DTCD- a chest specialist with more than 30 years of practice and a flair for writing clinical articles, Dr Kamal Kant Kohli joined Medical Dialogues as a Chief Editor of Medical News. Besides writing articles, as an editor, he proofreads and verifies all the medical content published on Medical Dialogues including those coming from journals, studies,medical conferences,guidelines etc. Email: drkohli@medicaldialogues.in. Contact no. 011-43720751