- Home
- Medical news & Guidelines
- Anesthesiology
- Cardiology and CTVS
- Critical Care
- Dentistry
- Dermatology
- Diabetes and Endocrinology
- ENT
- Gastroenterology
- Medicine
- Nephrology
- Neurology
- Obstretics-Gynaecology
- Oncology
- Ophthalmology
- Orthopaedics
- Pediatrics-Neonatology
- Psychiatry
- Pulmonology
- Radiology
- Surgery
- Urology
- Laboratory Medicine
- Diet
- Nursing
- Paramedical
- Physiotherapy
- Health news
- Fact Check
- Bone Health Fact Check
- Brain Health Fact Check
- Cancer Related Fact Check
- Child Care Fact Check
- Dental and oral health fact check
- Diabetes and metabolic health fact check
- Diet and Nutrition Fact Check
- Eye and ENT Care Fact Check
- Fitness fact check
- Gut health fact check
- Heart health fact check
- Kidney health fact check
- Medical education fact check
- Men's health fact check
- Respiratory fact check
- Skin and hair care fact check
- Vaccine and Immunization fact check
- Women's health fact check
- AYUSH
- State News
- Andaman and Nicobar Islands
- Andhra Pradesh
- Arunachal Pradesh
- Assam
- Bihar
- Chandigarh
- Chattisgarh
- Dadra and Nagar Haveli
- Daman and Diu
- Delhi
- Goa
- Gujarat
- Haryana
- Himachal Pradesh
- Jammu & Kashmir
- Jharkhand
- Karnataka
- Kerala
- Ladakh
- Lakshadweep
- Madhya Pradesh
- Maharashtra
- Manipur
- Meghalaya
- Mizoram
- Nagaland
- Odisha
- Puducherry
- Punjab
- Rajasthan
- Sikkim
- Tamil Nadu
- Telangana
- Tripura
- Uttar Pradesh
- Uttrakhand
- West Bengal
- Medical Education
- Industry
Combination of radiomics and machine learning can accurately predict pure uric acid urinary stones: Study
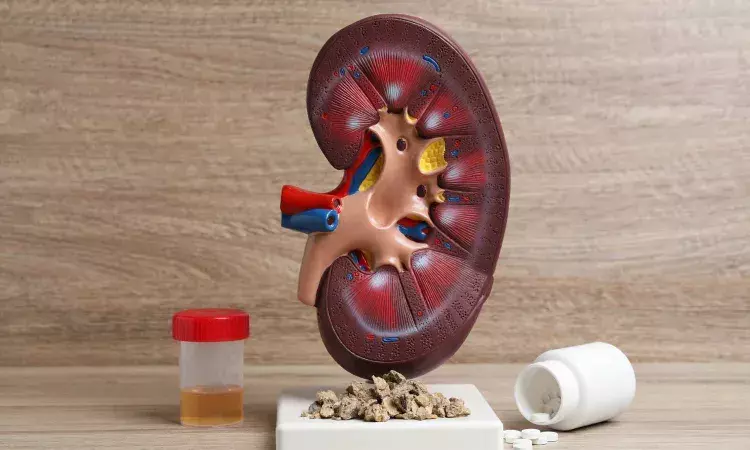
Korea: A recent study published in the World Journal of Urology has suggested a potential assisting tool for the treatment selection of stone disease.
The researchers revealed that a machine learning algorithm trained with radiomics features from non-contrast-enhanced computed tomography scans (NCCTs) accurately predicted pure uric acid (pUA) urinary stones.
Oral chemolysis is a non-invasive and effective treatment for uric acid urinary stones. Kyung-Jin Oh, Chonnam National University Medical School and Hospital, Gwangju, Korea, and colleagues aimed to classify urinary stones into pure uric acid or other composition (Others) using non-contrast-enhanced computed tomography scans.
For this purpose, the researchers screened instances managed at their institution from 2019 to 2021. They were labeled as pUA or Others based on composition analyses, and randomly split into training or testing data sets. Several instances that contained multiple NCCTs were collected. In each of the NCCTs, individual urinary stone was treated as individual samples.
The researchers extracted original and wavelet radiomic features for each sample from manually drawn volumes of interest. Then, the most important features were selected via the Least Absolute Shrinkage and Selection Operator for building the final model on a Support Vector Machine. Performance on the testing set was evaluated via sensitivity, accuracy, specificity, and area under the precision–recall curve (AUPRC).
The study led to the following findings:
- There were 302 instances, of which 118 had pUA urinary stones, generating 576 samples in total.
- From 851 original and wavelet radiomics features extracted for each sample, the 10 most important features were ultimately selected.
- On the testing data set, accuracy, sensitivity, specificity, and AUPRC was 93.9%, 97.9%, 92.2%, and 0.958, respectively, for per-sample prediction, and 90.8%, 100%, 87.5%, and 0.902, for per-instance prediction.
In conclusion, the machine learning algorithm trained with radiomics features from NCCTs can accurately predict pUA urinary stones. The work suggests a potential assisting tool for stone disease treatment selection.
Reference:
Le, B.D., Nguyen, T.A., Baek, B.H. et al. Accurate prediction of pure uric acid urinary stones in clinical context via a combination of radiomics and machine learning. World J Urol 42, 150 (2024). https://doi.org/10.1007/s00345-024-04818-4
Dr Kamal Kant Kohli-MBBS, DTCD- a chest specialist with more than 30 years of practice and a flair for writing clinical articles, Dr Kamal Kant Kohli joined Medical Dialogues as a Chief Editor of Medical News. Besides writing articles, as an editor, he proofreads and verifies all the medical content published on Medical Dialogues including those coming from journals, studies,medical conferences,guidelines etc. Email: drkohli@medicaldialogues.in. Contact no. 011-43720751