- Home
- Medical news & Guidelines
- Anesthesiology
- Cardiology and CTVS
- Critical Care
- Dentistry
- Dermatology
- Diabetes and Endocrinology
- ENT
- Gastroenterology
- Medicine
- Nephrology
- Neurology
- Obstretics-Gynaecology
- Oncology
- Ophthalmology
- Orthopaedics
- Pediatrics-Neonatology
- Psychiatry
- Pulmonology
- Radiology
- Surgery
- Urology
- Laboratory Medicine
- Diet
- Nursing
- Paramedical
- Physiotherapy
- Health news
- Fact Check
- Bone Health Fact Check
- Brain Health Fact Check
- Cancer Related Fact Check
- Child Care Fact Check
- Dental and oral health fact check
- Diabetes and metabolic health fact check
- Diet and Nutrition Fact Check
- Eye and ENT Care Fact Check
- Fitness fact check
- Gut health fact check
- Heart health fact check
- Kidney health fact check
- Medical education fact check
- Men's health fact check
- Respiratory fact check
- Skin and hair care fact check
- Vaccine and Immunization fact check
- Women's health fact check
- AYUSH
- State News
- Andaman and Nicobar Islands
- Andhra Pradesh
- Arunachal Pradesh
- Assam
- Bihar
- Chandigarh
- Chattisgarh
- Dadra and Nagar Haveli
- Daman and Diu
- Delhi
- Goa
- Gujarat
- Haryana
- Himachal Pradesh
- Jammu & Kashmir
- Jharkhand
- Karnataka
- Kerala
- Ladakh
- Lakshadweep
- Madhya Pradesh
- Maharashtra
- Manipur
- Meghalaya
- Mizoram
- Nagaland
- Odisha
- Puducherry
- Punjab
- Rajasthan
- Sikkim
- Tamil Nadu
- Telangana
- Tripura
- Uttar Pradesh
- Uttrakhand
- West Bengal
- Medical Education
- Industry
Study Unveils Biases: Machine Learning for Augmented Detection of Perinatal Mood and Anxiety Disorders
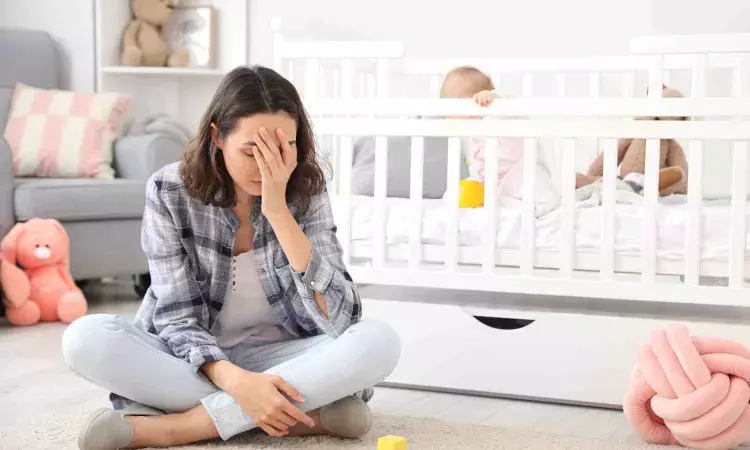
Recent research study focused on evaluating bias-mitigated predictive models of perinatal mood and anxiety disorders (PMADs) using machine learning for augmented screening. The study aimed to mitigate biases in predictive models trained on electronic health records (EHRs) data collected from 2020 to 2023 at Cedars-Sinai Medical Center in Los Angeles, California. The study included birthing patients aged 14 to 59 years with live birth records who were admitted to the postpartum unit or the maternal-fetal care unit after delivery.
Model Training and Evaluation
Patient-reported race and ethnicity data obtained from EHRs were used as exposure variables. Logistic regression, random forest, and extreme gradient boosting models were trained to predict moderate to high-risk (positive) screens using the Patient Health Questionnaire (PHQ-9) and the Edinburgh Postnatal Depression Scale (EPDS). The models were assessed with or without reweighing the data during preprocessing to evaluate bias mitigation and model performance.
Patient Analysis and Model Performance
Among the 19,430 patients in the study, racial and ethnic minority patients were more likely to screen positive for PMADs compared to non-Hispanic White patients. Models achieved modest performance with mean AUROCs ranging from 0.602 to 0.635 without reweighing and 0.602 to 0.622 with reweighing. Baseline models showed disparities in predicting postpartum depression, with reweighing reducing these differences in demographic parity and false-negative rates among racial and ethnic groups.
Addressing Health Disparities in Predictive Models
The study highlighted the importance of using target variables that are less likely to reflect existing disparities to prevent widening health disparities in PMAD diagnosis and treatment. Machine learning can augment traditional screening procedures to promote more equitable and routine PMAD screening. The study emphasized the need for model designs that integrate knowledge of health disparities to limit algorithmic bias and provide a nuanced understanding of potential biases in predictive models of PMAD.
Model Assessment and Bias Mitigation
The research included supervised classification algorithms, hyperparameter tuning, and repeated K-fold cross-validation to evaluate model performance and bias. Various fairness metrics were employed, such as demographic parity and false-negative rates, to assess biases across racial and ethnic groups. Methods like reweighing were used to minimize bias in the models, although deterministic reweighing based on frequencies may potentially introduce new biases against certain groups.
Study Findings and Recommendations
The study results demonstrated that the models did not perpetuate biases against racial and ethnic minorities relative to non-Hispanic White patients. The research recommended further exploration to optimize model weights to achieve specific performance and fairness goals. Acknowledging that machine learning alone cannot resolve all health disparities, the study proposed machine learning as a part of achieving more equitable mental health care, alongside restructuring clinical workflows and enhancing mental health services.
Key Points
-- The study aimed to evaluate bias-mitigated predictive models of perinatal mood and anxiety disorders (PMADs) using machine learning for augmented screening.
- Data collected from 2020 to 2023 at Cedars-Sinai Medical Center in Los Angeles, California, from birthing patients aged 14 to 59 years with live birth records were used for model training and evaluation.
- Patient-reported race and ethnicity data from electronic health records (EHRs) were utilized as exposure variables to predict moderate to high-risk PMAD screens using logistic regression, random forest, and extreme gradient boosting models.
- Racial and ethnic minority patients were more likely to screen positive for PMADs compared to non-Hispanic White patients, with models achieving modest performance in predicting PMAD risks.
- The study underscored the importance of using target variables less likely to reflect existing disparities to prevent widening health disparities in PMAD diagnosis and treatment.
- Various fairness metrics and bias mitigation techniques, such as reweighing, were employed to minimize biases in the predictive models, with recommendations for further exploration and optimization of model weights to achieve specific performance and fairness goals.
Reference –
Emily Wong et al. (2024). Evaluating Bias-Mitigated Predictive Models Of Perinatal Mood And Anxiety Disorders. * Emily Wong et al. (2024). Evaluating Bias-Mitigated Predictive Models Of Perinatal Mood And Anxiety Disorders. *JAMA Network Open*, 7. https://doi.org/10.1001/jamanetworkopen.2024.38152
Dr Kamal Kant Kohli-MBBS, DTCD- a chest specialist with more than 30 years of practice and a flair for writing clinical articles, Dr Kamal Kant Kohli joined Medical Dialogues as a Chief Editor of Medical News. Besides writing articles, as an editor, he proofreads and verifies all the medical content published on Medical Dialogues including those coming from journals, studies,medical conferences,guidelines etc. Email: drkohli@medicaldialogues.in. Contact no. 011-43720751