- Home
- Medical news & Guidelines
- Anesthesiology
- Cardiology and CTVS
- Critical Care
- Dentistry
- Dermatology
- Diabetes and Endocrinology
- ENT
- Gastroenterology
- Medicine
- Nephrology
- Neurology
- Obstretics-Gynaecology
- Oncology
- Ophthalmology
- Orthopaedics
- Pediatrics-Neonatology
- Psychiatry
- Pulmonology
- Radiology
- Surgery
- Urology
- Laboratory Medicine
- Diet
- Nursing
- Paramedical
- Physiotherapy
- Health news
- Fact Check
- Bone Health Fact Check
- Brain Health Fact Check
- Cancer Related Fact Check
- Child Care Fact Check
- Dental and oral health fact check
- Diabetes and metabolic health fact check
- Diet and Nutrition Fact Check
- Eye and ENT Care Fact Check
- Fitness fact check
- Gut health fact check
- Heart health fact check
- Kidney health fact check
- Medical education fact check
- Men's health fact check
- Respiratory fact check
- Skin and hair care fact check
- Vaccine and Immunization fact check
- Women's health fact check
- AYUSH
- State News
- Andaman and Nicobar Islands
- Andhra Pradesh
- Arunachal Pradesh
- Assam
- Bihar
- Chandigarh
- Chattisgarh
- Dadra and Nagar Haveli
- Daman and Diu
- Delhi
- Goa
- Gujarat
- Haryana
- Himachal Pradesh
- Jammu & Kashmir
- Jharkhand
- Karnataka
- Kerala
- Ladakh
- Lakshadweep
- Madhya Pradesh
- Maharashtra
- Manipur
- Meghalaya
- Mizoram
- Nagaland
- Odisha
- Puducherry
- Punjab
- Rajasthan
- Sikkim
- Tamil Nadu
- Telangana
- Tripura
- Uttar Pradesh
- Uttrakhand
- West Bengal
- Medical Education
- Industry
AI-screened eye pics diagnose autism with 100% accuracy
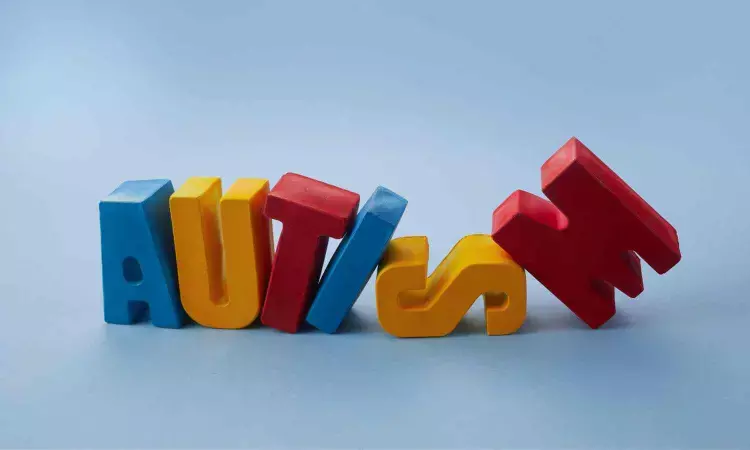
Retinal scans are advanced biometric techniques that scans retinal blood vessels and its different for each individdual. It is used for various purposes including security checks, however retinalscan as diagostic purpose has gained its importance.
Researchers have taken photographs of children’s retinas and screened them using a deep learning AI algorithm to diagnose autism with 100% accuracy. The findings support using AI as an objective screening tool for early diagnosis, especially when access to a specialist child psychiatrist is limited. The study was published in the journal JAMA Network Open.
At the back of the eye, the retina and the optic nerve connect at the optic disc. An extension of the central nervous system, the structure is a window into the brain and researchers have started capitalizing on their ability to easily and non-invasively access this body part to obtain important brain-related information.
Recently, UK researchers created a non-invasive means of rapidly diagnosing concussion by shining an eye-safe laser onto the retina. Now, researchers from Yonsei University College of Medicine in South Korea have developed a method of diagnosing autism spectrum disorder (ASD) and symptom severity in children using retinal images screened by an AI algorithm.
The researchers recruited 958 participants with a mean age of 7.8 years and photographed their retinas, resulting in a total of 1,890 images. Half of the participants had been diagnosed with ASD, and half were age- and sex-matched controls. ASD symptom severity was assessed using Autism Diagnostic Observation Schedule – Second Edition (ADOS-2) calibrated severity scores and Social Responsiveness Scale – Second Edition (SRS-2) scores.
A convolutional neural network, a deep learning algorithm, was trained using 85% of the retinal images and symptom severity test scores to construct models to screen for ASD and ASD symptom severity. The remaining 15% of images were retained for testing.
For ASD screening on the test set of images, the AI could pick out the children with an ASD diagnosis with a mean area under the receiver operating characteristic (AUROC) curve of 1.00. AUROC ranges in value from 0 to 1. A model whose predictions are 100% wrong has an AUROC of 0.0; one whose predictions are 100% correct has an AUROC of 1.0, indicating that the AI’s predictions in the current study were 100% correct. There was no notable decrease in the mean AUROC, even when 95% of the least important areas of the image – those not including the optic disc – were removed.
“Our models had promising performance in differentiating between ASD and TD [children with typical development] using retinal photographs, implying that retinal alterations in ASD may have potential value as biomarkers,” said the researchers. “Interestingly, these models retained a mean AUROC of 1.00 using only 10% of the image containing the optic disc, indicating that this area is crucial for distinguishing ASD from TD.”
The mean AUROC value for symptom severity was 0.74, where an AUROC of 0.7 to 0.8 is considered ‘acceptable’ and 0.8 to 0.9 is ‘excellent’.
“Our findings suggest that retinal photographs may provide additional information regarding symptom severity,” the researchers said. “We observed that feasible classification was achievable only for ADOS-2 scores and not for SRS-2 scores. This may be because the ADOS-2 is conducted by a trained professional with ample time for assessment, whereas the SRS-2 is typically completed by a caregiver in a few dozen minutes; thus, the former would reflect one’s severity status more accurately than the latter.”
Study participants were as young as four. Based on their findings, the researchers say that their AI-based model could be used as an objective screening tool from that age onwards. Because the newborn retina continues to grow until the age of four, further research is needed to ascertain whether the tool would be accurate for participants younger than that.
“Although future studies are required to establish generalizability, our study represents a notable step toward developing objective screening tools for ASD, which may help address urgent issues such as the inaccessibility of specialized child psychiatry assessments due to limited resources,” said the researchers.
Reference:
Kim JH, Hong J, Choi H, et al. Development of Deep Ensembles to Screen for Autism and Symptom Severity Using Retinal Photographs. JAMA Netw Open. 2023;6(12):e2347692. doi:10.1001/jamanetworkopen.2023.47692
MSc. Neuroscience
Niveditha Subramani a MSc. Neuroscience (Faculty of Medicine) graduate from University of Madras, Chennai. Ambitious in Neuro research having worked in motor diseases and neuron apoptosis is interested in more of new upcoming research and their advancement in field of medicine. She has an engrossed skill towards writing and her roles at Medical dialogue include Sr. Content writer. Her news covers new discoveries and updates in field of medicine. She can be reached at editorial@medicaldialogues.in
Dr Kamal Kant Kohli-MBBS, DTCD- a chest specialist with more than 30 years of practice and a flair for writing clinical articles, Dr Kamal Kant Kohli joined Medical Dialogues as a Chief Editor of Medical News. Besides writing articles, as an editor, he proofreads and verifies all the medical content published on Medical Dialogues including those coming from journals, studies,medical conferences,guidelines etc. Email: drkohli@medicaldialogues.in. Contact no. 011-43720751