- Home
- Medical news & Guidelines
- Anesthesiology
- Cardiology and CTVS
- Critical Care
- Dentistry
- Dermatology
- Diabetes and Endocrinology
- ENT
- Gastroenterology
- Medicine
- Nephrology
- Neurology
- Obstretics-Gynaecology
- Oncology
- Ophthalmology
- Orthopaedics
- Pediatrics-Neonatology
- Psychiatry
- Pulmonology
- Radiology
- Surgery
- Urology
- Laboratory Medicine
- Diet
- Nursing
- Paramedical
- Physiotherapy
- Health news
- Fact Check
- Bone Health Fact Check
- Brain Health Fact Check
- Cancer Related Fact Check
- Child Care Fact Check
- Dental and oral health fact check
- Diabetes and metabolic health fact check
- Diet and Nutrition Fact Check
- Eye and ENT Care Fact Check
- Fitness fact check
- Gut health fact check
- Heart health fact check
- Kidney health fact check
- Medical education fact check
- Men's health fact check
- Respiratory fact check
- Skin and hair care fact check
- Vaccine and Immunization fact check
- Women's health fact check
- AYUSH
- State News
- Andaman and Nicobar Islands
- Andhra Pradesh
- Arunachal Pradesh
- Assam
- Bihar
- Chandigarh
- Chattisgarh
- Dadra and Nagar Haveli
- Daman and Diu
- Delhi
- Goa
- Gujarat
- Haryana
- Himachal Pradesh
- Jammu & Kashmir
- Jharkhand
- Karnataka
- Kerala
- Ladakh
- Lakshadweep
- Madhya Pradesh
- Maharashtra
- Manipur
- Meghalaya
- Mizoram
- Nagaland
- Odisha
- Puducherry
- Punjab
- Rajasthan
- Sikkim
- Tamil Nadu
- Telangana
- Tripura
- Uttar Pradesh
- Uttrakhand
- West Bengal
- Medical Education
- Industry
ChatGPT has low diagnostic accuracy in pediatric cases, finds JAMA study
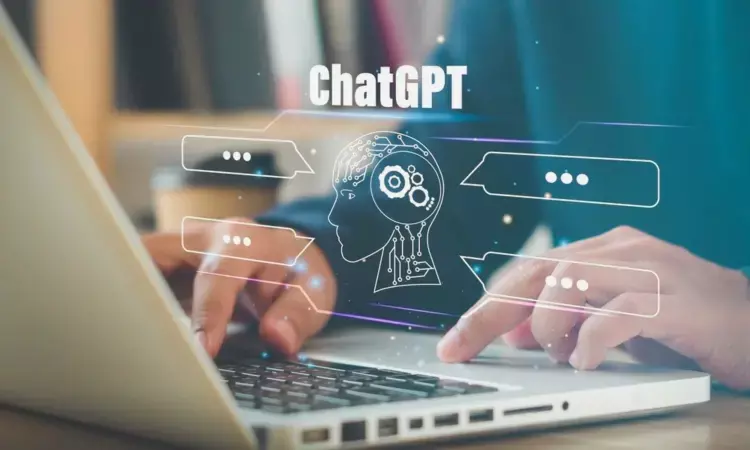
USA: A recent study published in JAMA Pediatrics has shed light on the diagnostic accuracy of a large language model (LLM) in pediatric case studies.
The researchers found that a LLM-based chatbot gave the wrong diagnosis for the majority of pediatric cases. They showed that the ChatGPT version 3.5 reached an incorrect diagnosis in 83 out of 100 pediatric case challenges. Among the incorrect diagnoses, 72 were incorrect, and 11 were clinically related to the correct diagnosis but too broad to be considered correct.
For example, ChatGPT got it wrong in a case of arthralgia and rash in a teenager with autism. The chatbot's diagnosis was "immune thrombocytopenic purpura" and the physician's diagnosis was "scurvy."
An example of an instance in which the chatbot diagnosis was determined to not fully capture the diagnosis was the case of a draining papule on the lateral neck of an infant. The chatbot diagnosis was "branchial cleft cyst" and the physician diagnosis was "branchio-oto-renal syndrome."
"Physicians should continue to investigate the applications of LLMs to medicine, despite the high error rate of the chatbot," Joseph Barile, Cohen Children’s Medical Center, New Hyde Park, New York, and colleagues wrote.
"Chatbots and LLMs have potential as an administrative tool for physicians, demonstrating proficiency in writing research articles and generating patient instructions."
A previous study investigating the diagnostic accuracy of ChatGPT version 4 found that the artificial intelligence (AI) chatbot rendered a correct diagnosis in 39% of New England Journal of Medicine (NEJM) case challenges. This suggested the use of LLM-based chatbots as a supplementary tool for clinicians in diagnosing and developing a differential list for complex cases.
"The capacity of large language models to process information and provide users with insights from vast amounts of data makes the technology well suited for algorithmic problem-solving," the researchers wrote.
According to the researchers, no research has explored the accuracy of LLM-based chatbots in solely pediatric scenarios, which need the consideration of the patient’s age alongside symptoms. Dr. Barile and colleagues assessed this accuracy across JAMA Pediatrics and NEJM pediatric case challenges.
For this purpose, the team pasted text from 100 cases into the ChatGPT version 3.5 with the following prompt: "List a differential diagnosis and a final diagnosis."
The chatbot-generated diagnoses were scored as "correct," "incorrect," or "did not fully capture diagnosis" by two physician researchers.
Barile and colleagues noted that more than half of the incorrect diagnoses generated by the chatbot belonged to the same organ system as the correct diagnosis. Additionally, 36% of the final case report diagnoses were included in the chatbot-generated differential list.
Reference:
Barile J, Margolis A, Cason G, et al. Diagnostic Accuracy of a Large Language Model in Pediatric Case Studies. JAMA Pediatr. Published online January 02, 2024. doi:10.1001/jamapediatrics.2023.5750
Dr Kamal Kant Kohli-MBBS, DTCD- a chest specialist with more than 30 years of practice and a flair for writing clinical articles, Dr Kamal Kant Kohli joined Medical Dialogues as a Chief Editor of Medical News. Besides writing articles, as an editor, he proofreads and verifies all the medical content published on Medical Dialogues including those coming from journals, studies,medical conferences,guidelines etc. Email: drkohli@medicaldialogues.in. Contact no. 011-43720751