- Home
- Medical news & Guidelines
- Anesthesiology
- Cardiology and CTVS
- Critical Care
- Dentistry
- Dermatology
- Diabetes and Endocrinology
- ENT
- Gastroenterology
- Medicine
- Nephrology
- Neurology
- Obstretics-Gynaecology
- Oncology
- Ophthalmology
- Orthopaedics
- Pediatrics-Neonatology
- Psychiatry
- Pulmonology
- Radiology
- Surgery
- Urology
- Laboratory Medicine
- Diet
- Nursing
- Paramedical
- Physiotherapy
- Health news
- Fact Check
- Bone Health Fact Check
- Brain Health Fact Check
- Cancer Related Fact Check
- Child Care Fact Check
- Dental and oral health fact check
- Diabetes and metabolic health fact check
- Diet and Nutrition Fact Check
- Eye and ENT Care Fact Check
- Fitness fact check
- Gut health fact check
- Heart health fact check
- Kidney health fact check
- Medical education fact check
- Men's health fact check
- Respiratory fact check
- Skin and hair care fact check
- Vaccine and Immunization fact check
- Women's health fact check
- AYUSH
- State News
- Andaman and Nicobar Islands
- Andhra Pradesh
- Arunachal Pradesh
- Assam
- Bihar
- Chandigarh
- Chattisgarh
- Dadra and Nagar Haveli
- Daman and Diu
- Delhi
- Goa
- Gujarat
- Haryana
- Himachal Pradesh
- Jammu & Kashmir
- Jharkhand
- Karnataka
- Kerala
- Ladakh
- Lakshadweep
- Madhya Pradesh
- Maharashtra
- Manipur
- Meghalaya
- Mizoram
- Nagaland
- Odisha
- Puducherry
- Punjab
- Rajasthan
- Sikkim
- Tamil Nadu
- Telangana
- Tripura
- Uttar Pradesh
- Uttrakhand
- West Bengal
- Medical Education
- Industry
Two views better than one in chest X-ray AI for classifying adenopathy: Study
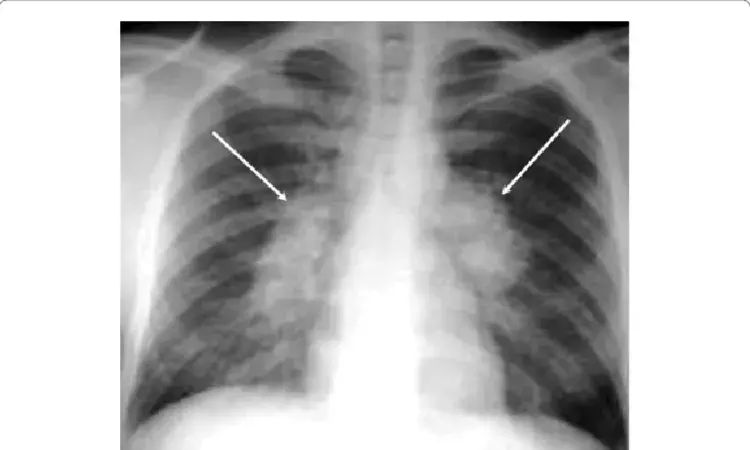
USA: A recent study has shown an artificial intelligence (AI) model, that analyzes both frontal and lateral, to be a better performer for classifying adenopathy. The study findings were presented Conference on Machine Intelligence in Medical Imaging (C-MIMI) on September 20, 2021.
Researchers from Johns Hopkins University found that the combined algorithm yielded the best results for classifying thoracic adenopathy. They had hypothesized that a deep-learning model that was trained on both frontal and lateral chest radiographs would perform better than a model that's trained on only one type of images.
Ishan Mazumdar, a second-year medical student said, "This is actually analogous to the common practice of radiologists who use both projections -- frontal and lateral -- to glean synergistic diagnostic details."
"This is actually analogous to the common practice of radiologists who use both projections -- frontal and lateral -- to glean synergistic diagnostic details," said presenter Ishan Mazumdar, a second-year medical student.
To test the hypothesis, they collected training and validation set from the PadChest dataset of radiographs, as well as Radiopedia for additional radiographs of hilar adenopathy specifically in patients with sarcoidosis. Ultimately, a training set of 2,107 paired frontal and lateral chest x-rays were used to train the models.
The researchers opted for a two-step deep convolutional neural network pipeline. In the first step, an object detection model was used to detect and crop the hilum on the radiographs. A hilum classification algorithm then classified the cropped image as having thoracic adenopathy or not.
They then tested the models on an external test set of 129 paired radiographs from patients at Johns Hopkins University who were diagnosed with sarcoidosis involving any organ. The test set had a higher prevalence of hilar adenopathy compared with the general population. For the purposes of the study, the ground truth was determined by board-certified thoracic radiologists after reviewing contemporaneous chest CT images.
The performance of each model was averaged over 10 testing "runs."
The researchers demonstrated an improvement in diagnostic accuracy when using deep-learning models trained using both frontal and lateral chest x-rays.
In addition to hilar adenopathy, other chest pathologies may also be better detected on the lateral projection, according to Mazumdar.
"Therefore, deep-learning models that ignore the lateral chest radiographs are not taking full advantage of commonly available data," he said.
Mazumdar acknowledged the limitations of their study, such as the inclusion in the training set of radiographs of patients with any type of adenopathy -- not just from sarcoidosis. This likely lowered the diagnostic performance of the algorithm, as the test set specifically included patients with sarcoidosis, he said.
Also, the ground truth for adenopathy is difficult to establish and subject to interobserver variability, and the optimal method of combining frontal and lateral chest x-rays isn't yet known, he said.
In the next phase of their work, the researchers plan to investigate different deep-learning methods for generating combined frontal and lateral predictions.
Dr Kamal Kant Kohli-MBBS, DTCD- a chest specialist with more than 30 years of practice and a flair for writing clinical articles, Dr Kamal Kant Kohli joined Medical Dialogues as a Chief Editor of Medical News. Besides writing articles, as an editor, he proofreads and verifies all the medical content published on Medical Dialogues including those coming from journals, studies,medical conferences,guidelines etc. Email: drkohli@medicaldialogues.in. Contact no. 011-43720751