- Home
- Medical news & Guidelines
- Anesthesiology
- Cardiology and CTVS
- Critical Care
- Dentistry
- Dermatology
- Diabetes and Endocrinology
- ENT
- Gastroenterology
- Medicine
- Nephrology
- Neurology
- Obstretics-Gynaecology
- Oncology
- Ophthalmology
- Orthopaedics
- Pediatrics-Neonatology
- Psychiatry
- Pulmonology
- Radiology
- Surgery
- Urology
- Laboratory Medicine
- Diet
- Nursing
- Paramedical
- Physiotherapy
- Health news
- Fact Check
- Bone Health Fact Check
- Brain Health Fact Check
- Cancer Related Fact Check
- Child Care Fact Check
- Dental and oral health fact check
- Diabetes and metabolic health fact check
- Diet and Nutrition Fact Check
- Eye and ENT Care Fact Check
- Fitness fact check
- Gut health fact check
- Heart health fact check
- Kidney health fact check
- Medical education fact check
- Men's health fact check
- Respiratory fact check
- Skin and hair care fact check
- Vaccine and Immunization fact check
- Women's health fact check
- AYUSH
- State News
- Andaman and Nicobar Islands
- Andhra Pradesh
- Arunachal Pradesh
- Assam
- Bihar
- Chandigarh
- Chattisgarh
- Dadra and Nagar Haveli
- Daman and Diu
- Delhi
- Goa
- Gujarat
- Haryana
- Himachal Pradesh
- Jammu & Kashmir
- Jharkhand
- Karnataka
- Kerala
- Ladakh
- Lakshadweep
- Madhya Pradesh
- Maharashtra
- Manipur
- Meghalaya
- Mizoram
- Nagaland
- Odisha
- Puducherry
- Punjab
- Rajasthan
- Sikkim
- Tamil Nadu
- Telangana
- Tripura
- Uttar Pradesh
- Uttrakhand
- West Bengal
- Medical Education
- Industry
Artificial intelligence could help reduce alcohol-related risks among surgery patients
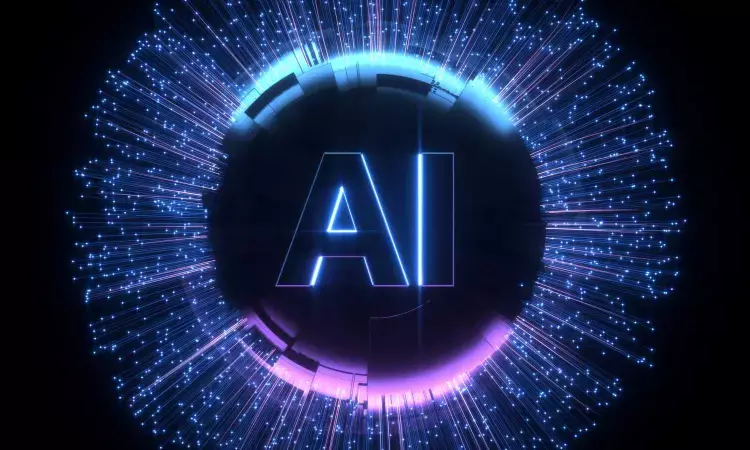
USA: A recent study showed that alcohol-related risk in patients' electronic health records (EHRs) can be efficiently and accurately identified with natural language processing (NLP), an artificial intelligence-based approach.
Using artificial intelligence (AI) to scan surgery patients’ medical records for signs of risky drinking might help spot those whose alcohol use raises their risk of problems during and after an operation, the study suggested.
The AI record scan tested in the study could help surgery teams know in advance which patients might need more education about such risks, or treatment to help them reduce their drinking or stop drinking for a period of time before and after surgery.
The findings, published in Alcohol: Clinical and Experimental Research by a team from the University of Michigan, show that using a form of AI called natural language processing to analyze a patient’s entire medical record can spot signs of risky drinking documented in their charts, such as in doctor’s notes, even when they don’t have a diagnosis of an alcohol problem.
Past research has shown that having more than a couple of drinks a day on average is associated with a higher risk of infections, wound complications, pulmonary complications and prolonged hospital stays in people having surgery.
Many people who drink regularly don’t have a problem with alcohol, and when they do they may never receive a formal diagnosis for alcohol use disorder or addiction, which would be easy for a surgical team to spot in their chart.
Scouring records and notes
The researchers, from Michigan Medicine, U-M’s academic medical center, trained their AI model by letting it review 100 anonymous surgical patients’ records to look for risky drinking signs, and comparing its classifications with those of expert human reviewers.
In all, the AI model matched the human expert classification most of the time. The AI model found signs of risky drinking in the notes of 87% of the patients who experts had identified as risky drinkers.
Meanwhile, only 29% of these patients had a diagnosis code related to alcohol in their list of diagnoses. So, many patients with higher risk for complications would have slipped under the radar for their surgical team.
The researchers then allowed the AI model to review more than 53,000 anonymous patient medical records compiled through the Michigan Genomics Initiative. The AI model identified three times more patients with risky alcohol use through this full-text search than the researchers found using diagnosis codes. In all, 15% of patients met criteria via the AI model, compared to 5% via diagnosis codes.
“This evaluation of natural language processing to identify risky drinking in the records of surgical patients could lay the groundwork for efforts to identify other risks in primary care and beyond, with appropriate validation,” said V. G. Vinod Vydiswaran, Ph.D., lead author of the new paper and an associate professor of learning health sciences at the U-M Medical School. “Essentially, this is a way of highlighting for a provider what is already contained in the notes made by other providers, without them having to read the entire record.”
“Given the excess surgical risk that can arise from even a moderate amount of daily alcohol use, and the challenges of implementing robust screening and treatment in the pre-op period, it’s vital that we explore other options for identifying patients who could most benefit from reducing use by themselves or with help, beyond those with a recorded diagnosis,” said senior author Anne Fernandez, Ph.D., an addiction psychologist at the U-M Addiction Center and Addiction Treatment Services and an associate professor of psychiatry.
The new data suggest that surgical clinics that simply review the diagnosis codes listed in their incoming patients’ charts, and flag ones such as alcohol use disorder, alcohol dependence or alcohol-related liver conditions, would be missing many patients with elevated risk.
Alcohol + surgery = added risk
In addition to known risks of surgical complications, Fernandez and colleagues recently published data from a massive Michigan surgical database showing that people who both smoke and have two or more drinks a day were more likely to end up back in the hospital, or back in the operating room, than others. Those with risky drinking who didn’t smoke also were more likely to need a second operation.
She and colleagues also found that 19% of people having surgery may have risky levels of alcohol use, in a review of detailed questionnaire data from people participating in two different studies that enroll people from Michigan Medicine surgery clinics.
The new study used the NLP form of AI not to generate new information, but to look for clues in the pages and pages of provider notes and data that make up a person’s entire medical record.
After validation, Vydiswaran said, the tool could potentially be run on a patient’s record before they are seen in a pre-operative appointment and identify their risk level.
Just knowing that a person has a potentially risky level of drinking isn’t enough, of course.
Fernandez is leading an effort to test a virtual coaching approach to help people scheduled for surgery understand the risks related to their level of drinking and support them in reducing their intake.
“Our goal is to identify people who may be in need of more treatment services, including medication for alcohol use disorder and support during their surgical recovery when alcohol abstinence is necessary,” she said. “We are not aiming to replace the due diligence every provider must do, but to prompt them to talk with patients and get more information to act upon.”
The risks of combining alcohol with the opioid pain medications often used to treat post-surgical pain are very high, she noted.
In addition to current work to validate the model, the team hopes to make their model publicly available, though it would have to be trained on the electronic records system of any health system that seeks to use it.
“These AI tools can do amazing things, but it’s important we use them to do things that could save time for busy clinicians, whether that’s related to alcohol or to drug use, disordered eating, or other chronic conditions,” said Fernandez. “And if we are going to use them to spot potential issues, we need to be ready to offer treatment options too."
Reference:
V. G. Vinod Vydiswaran, Asher Strayhorn, Katherine Weber, Haley Stevens, Jessica Mellinger, G. Scott Winder, Anne C. Fernandez, Automated-detection of risky alcohol use prior to surgery using natural language processing, Alcohol Clinical and Experimental Research, https://doi.org/10.1111/acer.15222.
Dr Kamal Kant Kohli-MBBS, DTCD- a chest specialist with more than 30 years of practice and a flair for writing clinical articles, Dr Kamal Kant Kohli joined Medical Dialogues as a Chief Editor of Medical News. Besides writing articles, as an editor, he proofreads and verifies all the medical content published on Medical Dialogues including those coming from journals, studies,medical conferences,guidelines etc. Email: drkohli@medicaldialogues.in. Contact no. 011-43720751