- Home
- Medical news & Guidelines
- Anesthesiology
- Cardiology and CTVS
- Critical Care
- Dentistry
- Dermatology
- Diabetes and Endocrinology
- ENT
- Gastroenterology
- Medicine
- Nephrology
- Neurology
- Obstretics-Gynaecology
- Oncology
- Ophthalmology
- Orthopaedics
- Pediatrics-Neonatology
- Psychiatry
- Pulmonology
- Radiology
- Surgery
- Urology
- Laboratory Medicine
- Diet
- Nursing
- Paramedical
- Physiotherapy
- Health news
- Fact Check
- Bone Health Fact Check
- Brain Health Fact Check
- Cancer Related Fact Check
- Child Care Fact Check
- Dental and oral health fact check
- Diabetes and metabolic health fact check
- Diet and Nutrition Fact Check
- Eye and ENT Care Fact Check
- Fitness fact check
- Gut health fact check
- Heart health fact check
- Kidney health fact check
- Medical education fact check
- Men's health fact check
- Respiratory fact check
- Skin and hair care fact check
- Vaccine and Immunization fact check
- Women's health fact check
- AYUSH
- State News
- Andaman and Nicobar Islands
- Andhra Pradesh
- Arunachal Pradesh
- Assam
- Bihar
- Chandigarh
- Chattisgarh
- Dadra and Nagar Haveli
- Daman and Diu
- Delhi
- Goa
- Gujarat
- Haryana
- Himachal Pradesh
- Jammu & Kashmir
- Jharkhand
- Karnataka
- Kerala
- Ladakh
- Lakshadweep
- Madhya Pradesh
- Maharashtra
- Manipur
- Meghalaya
- Mizoram
- Nagaland
- Odisha
- Puducherry
- Punjab
- Rajasthan
- Sikkim
- Tamil Nadu
- Telangana
- Tripura
- Uttar Pradesh
- Uttrakhand
- West Bengal
- Medical Education
- Industry
Machine Learning Model may help Identify High-Risk Surgical Patients Using electronic health records
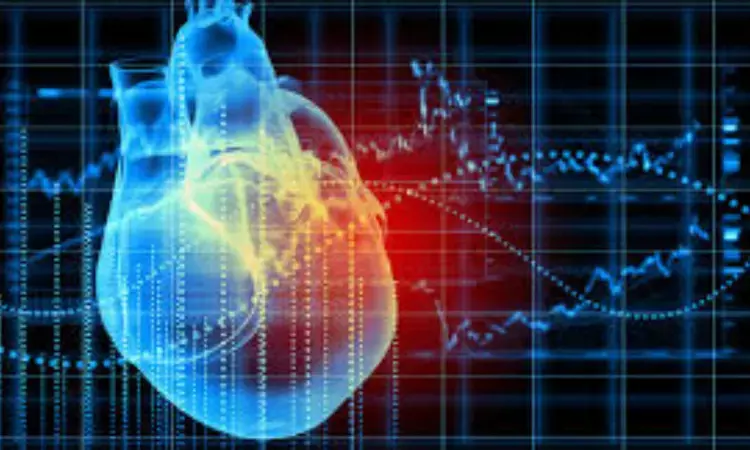
A recent study by Aman Mahajan and team at the University of Pittsburgh Medical Center (UPMC) has shown that an automated machine-learning model, utilizing data solely from electronic health records (EHRs), can accurately predict which patients are at a heightened risk of adverse outcomes from surgery. The findings were published in Journal of American Medical Association.
The study, which involved 1,477,561 patients undergoing surgery at 20 community and tertiary care hospitals within the UPMC health network, comprised three phases. Firstly, the model was built and validated using retrospective data, then its accuracy was tested on another set of retrospective data. Finally, the model was prospectively validated in clinical care.
The researchers employed a gradient-boosted decision tree machine learning method to develop the preoperative surgical risk prediction tool. To ensure interpretability and further validation of the model, the Shapley additive explanations method was used. The performance of the UPMC model was compared with the National Surgical Quality Improvement Program (NSQIP) surgical risk calculator in predicting mortality.
The results demonstrated the efficacy of the machine-learning model in identifying high-risk patients. The area under the receiver operating characteristic curve (AUROC) for mortality was 0.972 for the training set and 0.946 for the test set. In terms of major adverse cardiac and cerebrovascular events (MACCEs) and mortality, the AUROC was 0.923 for the training set and 0.899 for the test set. In the prospective evaluation, the AUROC for mortality was 0.956.
Furthermore, the model outperformed the NSQIP tool in terms of AUROC, specificity, and accuracy. The AUROC for the UPMC model was 0.945 compared to 0.897 for the NSQIP tool. The specificity and accuracy of the UPMC model were also superior, with values of 0.87 and 0.85, respectively, compared to 0.68 and 0.69 for the NSQIP tool.
The findings of this study hold significant implications for improving surgical outcomes. By accurately identifying patients at high risk of adverse events using only preoperative variables from EHRs, clinicians can provide individualized perioperative care and interventions. This tailored approach has the potential to enhance patient outcomes and reduce the incidence of adverse events associated with surgery.
Source:
Mahajan, A., Esper, S., Oo, T. H., McKibben, J., Garver, M., Artman, J., Klahre, C., Ryan, J., Sadhasivam, S., Holder-Murray, J., & Marroquin, O. C. (2023). Development and Validation of a Machine Learning Model to Identify Patients Before Surgery at High Risk for Postoperative Adverse Events. In JAMA Network Open. American Medical Association (AMA). https://doi.org/10.1001/jamanetworkopen.2023.22285
Neuroscience Masters graduate
Jacinthlyn Sylvia, a Neuroscience Master's graduate from Chennai has worked extensively in deciphering the neurobiology of cognition and motor control in aging. She also has spread-out exposure to Neurosurgery from her Bachelor’s. She is currently involved in active Neuro-Oncology research. She is an upcoming neuroscientist with a fiery passion for writing. Her news cover at Medical Dialogues feature recent discoveries and updates from the healthcare and biomedical research fields. She can be reached at editorial@medicaldialogues.in
Dr Kamal Kant Kohli-MBBS, DTCD- a chest specialist with more than 30 years of practice and a flair for writing clinical articles, Dr Kamal Kant Kohli joined Medical Dialogues as a Chief Editor of Medical News. Besides writing articles, as an editor, he proofreads and verifies all the medical content published on Medical Dialogues including those coming from journals, studies,medical conferences,guidelines etc. Email: drkohli@medicaldialogues.in. Contact no. 011-43720751