- Home
- Medical news & Guidelines
- Anesthesiology
- Cardiology and CTVS
- Critical Care
- Dentistry
- Dermatology
- Diabetes and Endocrinology
- ENT
- Gastroenterology
- Medicine
- Nephrology
- Neurology
- Obstretics-Gynaecology
- Oncology
- Ophthalmology
- Orthopaedics
- Pediatrics-Neonatology
- Psychiatry
- Pulmonology
- Radiology
- Surgery
- Urology
- Laboratory Medicine
- Diet
- Nursing
- Paramedical
- Physiotherapy
- Health news
- Fact Check
- Bone Health Fact Check
- Brain Health Fact Check
- Cancer Related Fact Check
- Child Care Fact Check
- Dental and oral health fact check
- Diabetes and metabolic health fact check
- Diet and Nutrition Fact Check
- Eye and ENT Care Fact Check
- Fitness fact check
- Gut health fact check
- Heart health fact check
- Kidney health fact check
- Medical education fact check
- Men's health fact check
- Respiratory fact check
- Skin and hair care fact check
- Vaccine and Immunization fact check
- Women's health fact check
- AYUSH
- State News
- Andaman and Nicobar Islands
- Andhra Pradesh
- Arunachal Pradesh
- Assam
- Bihar
- Chandigarh
- Chattisgarh
- Dadra and Nagar Haveli
- Daman and Diu
- Delhi
- Goa
- Gujarat
- Haryana
- Himachal Pradesh
- Jammu & Kashmir
- Jharkhand
- Karnataka
- Kerala
- Ladakh
- Lakshadweep
- Madhya Pradesh
- Maharashtra
- Manipur
- Meghalaya
- Mizoram
- Nagaland
- Odisha
- Puducherry
- Punjab
- Rajasthan
- Sikkim
- Tamil Nadu
- Telangana
- Tripura
- Uttar Pradesh
- Uttrakhand
- West Bengal
- Medical Education
- Industry
Revolutionizing Glioma Care: Machine Learning and fMRI Predict Post-Surgical Functional Status, study finds
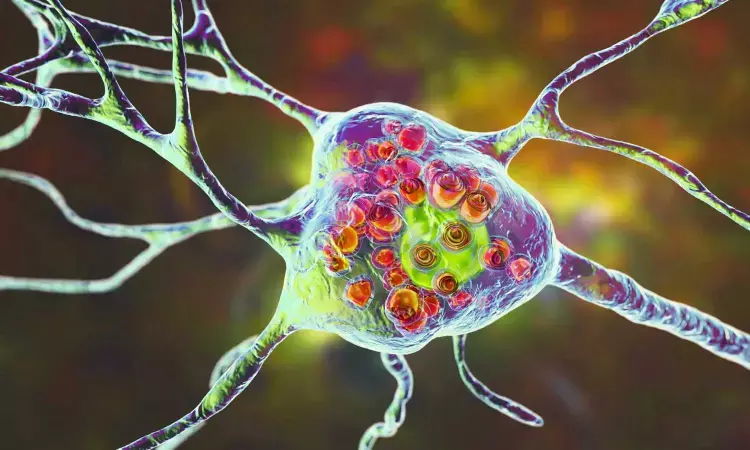
USA: In a groundbreaking leap forward for neuro-oncology, a recent study has unveiled a pioneering approach to predicting post-surgical functional status in high-grade glioma (HGG) patients using resting-state functional magnetic resonance imaging (fMRI) and machine learning algorithms. The research heralds a transformative era in personalized treatment strategies for one of the most aggressive forms of brain cancer.
Researchers found that a machine-learning algorithm with resting-state functional MRI can help clinicians foresee surgical outcomes in high-grade glioma patients. They developed a random-forest classifier that was highly accurate for predicting tumor resection outcomes in brain cancer patients.
The findings were published online in the Journal of Neuro-Oncology on 24 May 2024.
"The capability to forecast postsurgical functional outcomes from the initial diagnosis could be advantageous in surgical planning and for better-informing patients of their likely treatment outcomes," the group noted.
High-grade gliomas, characterized by their rapid growth and invasive nature, present formidable challenges for clinicians tasked with navigating the delicate balance between tumor resection and preserving neurological function. Traditional methods for assessing postoperative functional status have often relied on subjective evaluations and empirical observations, leaving room for uncertainty and variability in treatment outcomes.
Patrick H. Luckett, Department of Neurological Surgery, Washington University School of Medicine, St. Louis, MO, USA, and colleagues aimed to develop models capable of predicting functional outcomes in HGG patients before surgery, facilitating improved disease management and informed patient care.
For this purpose, the research team retrospectively recruited adult HGG patients (N = 102) from the neurosurgery brain tumor service at Washington University Medical Center. All patients completed resting-state functional MRI and structural neuroimaging before surgery.
Demographics, tumor location, measures of resting state network connectivity (FC), and tumor volume were used to train a random forest classifier to predict functional outcomes based on Karnofsky Performance Status (KPS < 70, KPS ≥ 70).
The following were the key findings of the study:
· The models achieved a nested cross-validation accuracy of 94.1% and an AUC of 0.97 in classifying KPS.
· The strongest predictors identified by the model included FC between somatomotor, visual, auditory, and reward networks.
· Based on location, the relation of the tumor to dorsal attention, cingulo-opercular, and basal ganglia networks were strong predictors of KPS.
· Age was also a strong predictor. However, tumor volume was only a moderate predictor.
The research demonstrates how machine learning can accurately classify HGG patients' functional outcomes before surgical, chemical, or radiotherapy treatments. These results were achieved using tumor location, age, tumor size, and RS-fMRI measures.
"By incorporating these models into clinical practice, we stand to enhance patient care, enabling personalized treatment plans that balance quality of life with survival," the researchers wrote. "Such models can drive a more nuanced approach to HGG patient management, prioritizing longevity and post-treatment quality of life."
Reference:
Luckett, P.H., Olufawo, M.O., Park, K.Y. et al. Predicting post-surgical functional status in high-grade glioma with resting-state fMRI and machine learning. J Neurooncol (2024). https://doi.org/10.1007/s11060-024-04715-1
MSc. Biotechnology
Medha Baranwal joined Medical Dialogues as an Editor in 2018 for Speciality Medical Dialogues. She covers several medical specialties including Cardiac Sciences, Dentistry, Diabetes and Endo, Diagnostics, ENT, Gastroenterology, Neurosciences, and Radiology. She has completed her Bachelors in Biomedical Sciences from DU and then pursued Masters in Biotechnology from Amity University. She has a working experience of 5 years in the field of medical research writing, scientific writing, content writing, and content management. She can be contacted at  editorial@medicaldialogues.in. Contact no. 011-43720751
Dr Kamal Kant Kohli-MBBS, DTCD- a chest specialist with more than 30 years of practice and a flair for writing clinical articles, Dr Kamal Kant Kohli joined Medical Dialogues as a Chief Editor of Medical News. Besides writing articles, as an editor, he proofreads and verifies all the medical content published on Medical Dialogues including those coming from journals, studies,medical conferences,guidelines etc. Email: drkohli@medicaldialogues.in. Contact no. 011-43720751