- Home
- Medical news & Guidelines
- Anesthesiology
- Cardiology and CTVS
- Critical Care
- Dentistry
- Dermatology
- Diabetes and Endocrinology
- ENT
- Gastroenterology
- Medicine
- Nephrology
- Neurology
- Obstretics-Gynaecology
- Oncology
- Ophthalmology
- Orthopaedics
- Pediatrics-Neonatology
- Psychiatry
- Pulmonology
- Radiology
- Surgery
- Urology
- Laboratory Medicine
- Diet
- Nursing
- Paramedical
- Physiotherapy
- Health news
- Fact Check
- Bone Health Fact Check
- Brain Health Fact Check
- Cancer Related Fact Check
- Child Care Fact Check
- Dental and oral health fact check
- Diabetes and metabolic health fact check
- Diet and Nutrition Fact Check
- Eye and ENT Care Fact Check
- Fitness fact check
- Gut health fact check
- Heart health fact check
- Kidney health fact check
- Medical education fact check
- Men's health fact check
- Respiratory fact check
- Skin and hair care fact check
- Vaccine and Immunization fact check
- Women's health fact check
- AYUSH
- State News
- Andaman and Nicobar Islands
- Andhra Pradesh
- Arunachal Pradesh
- Assam
- Bihar
- Chandigarh
- Chattisgarh
- Dadra and Nagar Haveli
- Daman and Diu
- Delhi
- Goa
- Gujarat
- Haryana
- Himachal Pradesh
- Jammu & Kashmir
- Jharkhand
- Karnataka
- Kerala
- Ladakh
- Lakshadweep
- Madhya Pradesh
- Maharashtra
- Manipur
- Meghalaya
- Mizoram
- Nagaland
- Odisha
- Puducherry
- Punjab
- Rajasthan
- Sikkim
- Tamil Nadu
- Telangana
- Tripura
- Uttar Pradesh
- Uttrakhand
- West Bengal
- Medical Education
- Industry
AI-Powered ECG Model Shows Promise in Heart Failure Risk Prediction: JAMA
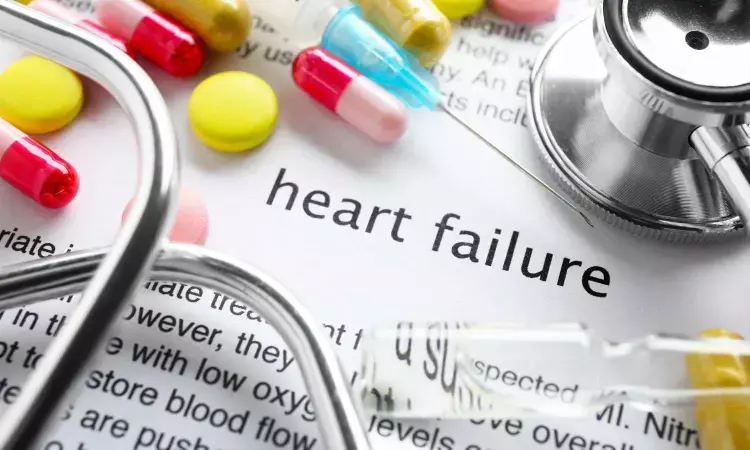
A new study published in the Journal of American Medical Association showed that a noise-adapted AI-ECG model successfully estimated heart failure risk using only lead I ECGs across diverse multinational cohorts. This suggests a potential strategy for heart failure (HF) risk stratification that could be applied using wearable and portable ECG devices, warranting further prospective studies.
Single-lead electrocardiograms (ECGs) may be recorded using portable equipment, which might allow for extensive community-based risk assessment. Thus, to determine if an artificial intelligence (AI) system can predict heart failure risk from noisy single-lead electrocardiograms, Lovedeep Dhingra and colleagues carried out this investigation.
A retrospective cohort analysis assessed persons without heart failure at baseline from UK Biobank, YNHHS, and ELSA-Brasil, utilizing outpatient ECG data. The data were evaluated between September 2023 and February 2025, with the major exposure being the AI-ECG-predicted risk of left ventricular systolic dysfunction (LVSD).
Lead I ECGs were separated to mimic wearable device signals, and a noise-adaptive AI-ECG model trained to identify LVSD was used. The model's relationship with new-onset heart failure (first HF hospitalization) was investigated. Its predictive ability was compared to the PREVENT and PCP-HF risk scores using the integrated discrimination improvement, Harrell C statistic, and net reclassification improvement.
Baseline ECGs were obtained from 192 667 YNHHS patients, 42 141 UKB participants, and 13 454 ELSA-Brasil participants. 31 (0.2%) in ELSA-Brasil, 46 (0.1%) in UKB, and 3697 (1.9%) in YNHHS experienced heart failure over a median (IQR) of 4.2 (3.7-4.5) years, 3.1 (2.1-4.5) years, and 4.6 (2.8-6.6) years, respectively.
Regardless of age, sex, comorbidities, or competing risk of mortality, a positive AI-ECG screening result for LVSD was linked to a 3- to 7-fold increased risk for HF, and every 0.1 increase in the model likelihood was linked to a 27% to 65% greater hazard across cohorts. The discrimination of AI-ECG for new-onset HF was 0.828 in ELSA-Brasil, 0.723 in YNHHS, and 0.736 in UKB.
Across cohorts, integrating AI-ECG predictions beside PCP-HF and PREVENT equations resulted in a higher Harrel C statistic. AI-ECG improved integrated discrimination by 0.091 to 0.205 vs PCP-HF and 0.068 to 0.192 vs PREVENT, as well as net reclassification by 18.2% to 47.2% vs PCP-HF and 11.8% to 47.5% vs PREVENT.
Overall, a noise-adapted AI-ECG model predicted HF risk using lead I ECGs across global cohorts, indicating a viable HF risk-stratification technique that needs to be studied prospectively employing wearable and portable ECG devices.
Source:
Dhingra, L. S., Aminorroaya, A., Pedroso, A. F., Khunte, A., Sangha, V., McIntyre, D., Chow, C. K., Asselbergs, F. W., Brant, L. C. C., Barreto, S. M., Ribeiro, A. L. P., Krumholz, H. M., Oikonomou, E. K., & Khera, R. (2025). Artificial intelligence-enabled prediction of heart failure risk from single-lead electrocardiograms. JAMA Cardiology. https://doi.org/10.1001/jamacardio.2025.0492
Neuroscience Masters graduate
Jacinthlyn Sylvia, a Neuroscience Master's graduate from Chennai has worked extensively in deciphering the neurobiology of cognition and motor control in aging. She also has spread-out exposure to Neurosurgery from her Bachelor’s. She is currently involved in active Neuro-Oncology research. She is an upcoming neuroscientist with a fiery passion for writing. Her news cover at Medical Dialogues feature recent discoveries and updates from the healthcare and biomedical research fields. She can be reached at editorial@medicaldialogues.in
Dr Kamal Kant Kohli-MBBS, DTCD- a chest specialist with more than 30 years of practice and a flair for writing clinical articles, Dr Kamal Kant Kohli joined Medical Dialogues as a Chief Editor of Medical News. Besides writing articles, as an editor, he proofreads and verifies all the medical content published on Medical Dialogues including those coming from journals, studies,medical conferences,guidelines etc. Email: drkohli@medicaldialogues.in. Contact no. 011-43720751