- Home
- Medical news & Guidelines
- Anesthesiology
- Cardiology and CTVS
- Critical Care
- Dentistry
- Dermatology
- Diabetes and Endocrinology
- ENT
- Gastroenterology
- Medicine
- Nephrology
- Neurology
- Obstretics-Gynaecology
- Oncology
- Ophthalmology
- Orthopaedics
- Pediatrics-Neonatology
- Psychiatry
- Pulmonology
- Radiology
- Surgery
- Urology
- Laboratory Medicine
- Diet
- Nursing
- Paramedical
- Physiotherapy
- Health news
- Fact Check
- Bone Health Fact Check
- Brain Health Fact Check
- Cancer Related Fact Check
- Child Care Fact Check
- Dental and oral health fact check
- Diabetes and metabolic health fact check
- Diet and Nutrition Fact Check
- Eye and ENT Care Fact Check
- Fitness fact check
- Gut health fact check
- Heart health fact check
- Kidney health fact check
- Medical education fact check
- Men's health fact check
- Respiratory fact check
- Skin and hair care fact check
- Vaccine and Immunization fact check
- Women's health fact check
- AYUSH
- State News
- Andaman and Nicobar Islands
- Andhra Pradesh
- Arunachal Pradesh
- Assam
- Bihar
- Chandigarh
- Chattisgarh
- Dadra and Nagar Haveli
- Daman and Diu
- Delhi
- Goa
- Gujarat
- Haryana
- Himachal Pradesh
- Jammu & Kashmir
- Jharkhand
- Karnataka
- Kerala
- Ladakh
- Lakshadweep
- Madhya Pradesh
- Maharashtra
- Manipur
- Meghalaya
- Mizoram
- Nagaland
- Odisha
- Puducherry
- Punjab
- Rajasthan
- Sikkim
- Tamil Nadu
- Telangana
- Tripura
- Uttar Pradesh
- Uttrakhand
- West Bengal
- Medical Education
- Industry
Machine Learning may predict Treatment outcomes in early childhood caries, suggests study
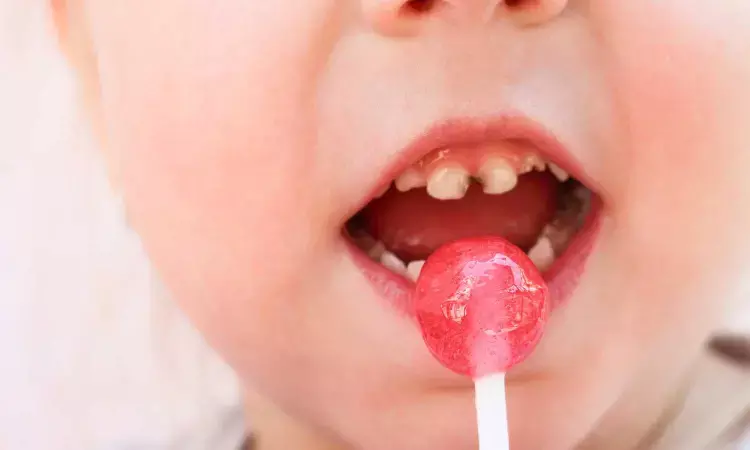
Researchers have found in a new study that Machine learning shows promise in predicting treatment outcomes in early childhood caries. Further Key identified predictors can help guide targeted management strategies.
Early childhood caries (ECC) is a major oral health problem among preschool children that can significantly influence children’s quality of life. Machine learning can accurately predict the treatment outcome but its use in ECC management is limited.
The aim of this study is to explore the application of machine learning in predicting the treatment outcome of ECC. This study was a secondary analysis of a recently published clinical trial that recruited 1,070 children aged 3- to 4-year-old with ECC. Machine learning algorithms including Naive Bayes, logistic regression, decision tree, random forest, support vector machine, and extreme gradient boosting were adopted to predict the caries-arresting outcome of ECC at 30-month follow-up after receiving fluoride and silver therapy.
Candidate predictors included clinical parameters (caries experience and oral hygiene status), oral health-related behaviours (toothbrushing habits, feeding history and snacking preference) and socioeconomic backgrounds of the children. Model performance was evaluated using discrimination and calibration metrics including accuracy, recall, precision, F1 score, area under the receiver operating characteristic curve (AUROC) and Brier score. Shapley additive explanations were deployed to identify the important predictors. Results: All machine learning models showed good performance in predicting the treatment outcome of ECC.
The accuracy, recall, precision, F1 score, AUROC, and Brier score of the six models ranged from 0.674 to 0.740, 0.731 to 0.809, 0.762 to 0.802, 0.741 to 0.804, 0.771 to 0.859, and 0.134 to 0.227, respectively. The important predictors of the caries-arresting outcome were the surface and tooth location of the carious lesions, newly developed caries during follow-ups, baseline caries experience, whether the children had assisted toothbrushing and oral hygiene status.
Machine learning can provide promising predictions of the treatment outcome of ECC. The identified key predictors would be particularly informative for targeted management of ECC.
Reference:
Wu, Y., Jia, M., Fang, Y. et al. Use machine learning to predict treatment outcome of early childhood caries. BMC Oral Health 25, 389 (2025). https://doi.org/10.1186/s12903-025-05768-y
Dr. Shravani Dali has completed her BDS from Pravara institute of medical sciences, loni. Following which she extensively worked in the healthcare sector for 2+ years. She has been actively involved in writing blogs in field of health and wellness. Currently she is pursuing her Masters of public health-health administration from Tata institute of social sciences. She can be contacted at editorial@medicaldialogues.in.
Dr Kamal Kant Kohli-MBBS, DTCD- a chest specialist with more than 30 years of practice and a flair for writing clinical articles, Dr Kamal Kant Kohli joined Medical Dialogues as a Chief Editor of Medical News. Besides writing articles, as an editor, he proofreads and verifies all the medical content published on Medical Dialogues including those coming from journals, studies,medical conferences,guidelines etc. Email: drkohli@medicaldialogues.in. Contact no. 011-43720751