- Home
- Medical news & Guidelines
- Anesthesiology
- Cardiology and CTVS
- Critical Care
- Dentistry
- Dermatology
- Diabetes and Endocrinology
- ENT
- Gastroenterology
- Medicine
- Nephrology
- Neurology
- Obstretics-Gynaecology
- Oncology
- Ophthalmology
- Orthopaedics
- Pediatrics-Neonatology
- Psychiatry
- Pulmonology
- Radiology
- Surgery
- Urology
- Laboratory Medicine
- Diet
- Nursing
- Paramedical
- Physiotherapy
- Health news
- Fact Check
- Bone Health Fact Check
- Brain Health Fact Check
- Cancer Related Fact Check
- Child Care Fact Check
- Dental and oral health fact check
- Diabetes and metabolic health fact check
- Diet and Nutrition Fact Check
- Eye and ENT Care Fact Check
- Fitness fact check
- Gut health fact check
- Heart health fact check
- Kidney health fact check
- Medical education fact check
- Men's health fact check
- Respiratory fact check
- Skin and hair care fact check
- Vaccine and Immunization fact check
- Women's health fact check
- AYUSH
- State News
- Andaman and Nicobar Islands
- Andhra Pradesh
- Arunachal Pradesh
- Assam
- Bihar
- Chandigarh
- Chattisgarh
- Dadra and Nagar Haveli
- Daman and Diu
- Delhi
- Goa
- Gujarat
- Haryana
- Himachal Pradesh
- Jammu & Kashmir
- Jharkhand
- Karnataka
- Kerala
- Ladakh
- Lakshadweep
- Madhya Pradesh
- Maharashtra
- Manipur
- Meghalaya
- Mizoram
- Nagaland
- Odisha
- Puducherry
- Punjab
- Rajasthan
- Sikkim
- Tamil Nadu
- Telangana
- Tripura
- Uttar Pradesh
- Uttrakhand
- West Bengal
- Medical Education
- Industry
Machine learning based pure-tone audiometry effective in diagnosing Meniere's disease: Study
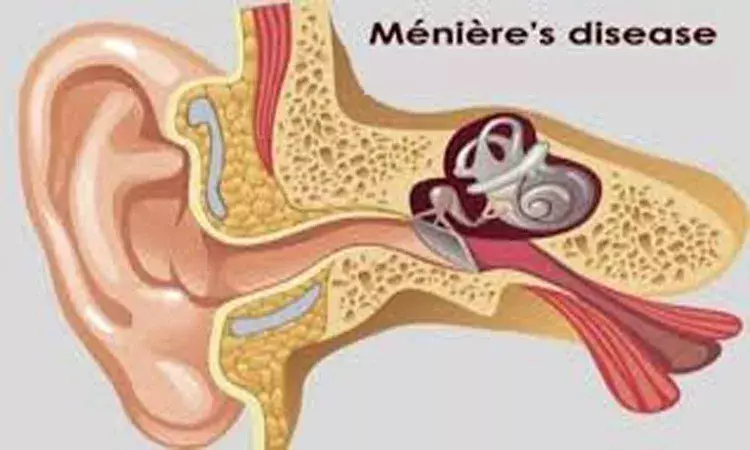
A new study published in the journal of Otolaryngology-Head and Neck Surgery showed that an effective machine learning model utilizing pure-tone audiometry characteristics effectively diagnosed Meniere's disease (MD) with the ability to foresee the subtypes of endolymphatic hydrops.
One of the most complicated disorders in otolaryngology is Meniere's disease which is a complex vestibular dysfunction that presents significant diagnostic hurdles. As of now, the etiology of MD is complex and poorly understood with endolymphatic hydrops (EH) as a key pathohistological characteristic. Even though EH may presently be seen using gadolinium-enhanced MRI, clinical symptoms still play a major role in the diagnosis of MD.
When MD is first diagnosed, it is frequently divided into "definite MD" and "probable MD." Thus, Xu Liu and team conducted this study with the purpose to deploy machine learning models for the automated diagnosis of Meniere's illness and the prediction of endolymphatic hydrops, based on the air conduction thresholds of pure-tone audiometry.
Pure-tone audiometry data and gadolinium-enhanced magnetic resonance imaging sequences were gathered. Based on the air conduction thresholds of pure-tone audiometry, basic and various analytical characteristics were then constructed. Afterwards, the constructed characteristics were used to train 5 traditional machine learning models for MD diagnosis. The models that performed exceptionally well were also chosen to forecast EH. The performance of the model in diagnosing MDs was evaluated against that of skilled otolaryngologists.
With an accuracy rate of 87%, sensitivity of 83%, specificity of 90%, and a strong area under the receiver operating characteristic curve of 0.95, the winning light gradient boosting (LGB) machine learning model trained by multiple features performs remarkably well on the diagnosis of MD.
Also, the LGB model was better than the other 3 machine learning models, with an accuracy of 78% on EH prediction. Further, a feature significance analysis highlights the critical relevance of certain pure-tone audiometry parameters that are necessary for EH prediction and MD diagnosis. At 250 Hz, the standard deviation and mean of the whole-frequency hearing, hearing at low frequencies and the peak audiogram stood out. Overall, this study supports the use of pure-tone audiometry data in an AI-based method to distinguish between MD.
Reference:
Liu, X., Guo, P., Wang, D., Hsieh, Y., Shi, S., Dai, Z., Wang, D., Li, H., & Wang, W. (2024). Applications of Machine Learning in Meniere’s Disease Assessment Based on Pure‐Tone Audiometry. In Otolaryngology–Head and Neck Surgery. Wiley. https://doi.org/10.1002/ohn.956
Neuroscience Masters graduate
Jacinthlyn Sylvia, a Neuroscience Master's graduate from Chennai has worked extensively in deciphering the neurobiology of cognition and motor control in aging. She also has spread-out exposure to Neurosurgery from her Bachelor’s. She is currently involved in active Neuro-Oncology research. She is an upcoming neuroscientist with a fiery passion for writing. Her news cover at Medical Dialogues feature recent discoveries and updates from the healthcare and biomedical research fields. She can be reached at editorial@medicaldialogues.in
Dr Kamal Kant Kohli-MBBS, DTCD- a chest specialist with more than 30 years of practice and a flair for writing clinical articles, Dr Kamal Kant Kohli joined Medical Dialogues as a Chief Editor of Medical News. Besides writing articles, as an editor, he proofreads and verifies all the medical content published on Medical Dialogues including those coming from journals, studies,medical conferences,guidelines etc. Email: drkohli@medicaldialogues.in. Contact no. 011-43720751