- Home
- Medical news & Guidelines
- Anesthesiology
- Cardiology and CTVS
- Critical Care
- Dentistry
- Dermatology
- Diabetes and Endocrinology
- ENT
- Gastroenterology
- Medicine
- Nephrology
- Neurology
- Obstretics-Gynaecology
- Oncology
- Ophthalmology
- Orthopaedics
- Pediatrics-Neonatology
- Psychiatry
- Pulmonology
- Radiology
- Surgery
- Urology
- Laboratory Medicine
- Diet
- Nursing
- Paramedical
- Physiotherapy
- Health news
- Fact Check
- Bone Health Fact Check
- Brain Health Fact Check
- Cancer Related Fact Check
- Child Care Fact Check
- Dental and oral health fact check
- Diabetes and metabolic health fact check
- Diet and Nutrition Fact Check
- Eye and ENT Care Fact Check
- Fitness fact check
- Gut health fact check
- Heart health fact check
- Kidney health fact check
- Medical education fact check
- Men's health fact check
- Respiratory fact check
- Skin and hair care fact check
- Vaccine and Immunization fact check
- Women's health fact check
- AYUSH
- State News
- Andaman and Nicobar Islands
- Andhra Pradesh
- Arunachal Pradesh
- Assam
- Bihar
- Chandigarh
- Chattisgarh
- Dadra and Nagar Haveli
- Daman and Diu
- Delhi
- Goa
- Gujarat
- Haryana
- Himachal Pradesh
- Jammu & Kashmir
- Jharkhand
- Karnataka
- Kerala
- Ladakh
- Lakshadweep
- Madhya Pradesh
- Maharashtra
- Manipur
- Meghalaya
- Mizoram
- Nagaland
- Odisha
- Puducherry
- Punjab
- Rajasthan
- Sikkim
- Tamil Nadu
- Telangana
- Tripura
- Uttar Pradesh
- Uttrakhand
- West Bengal
- Medical Education
- Industry
New Biomarkers Promising for Early Prediction of Gestational Diabetes: Study Reveals
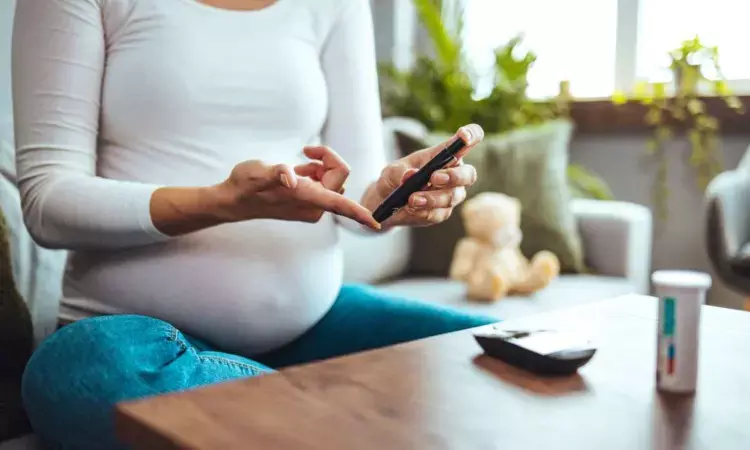
China: Recent research has unveiled promising findings in predicting gestational diabetes mellitus (GDM) by analyzing multiple biomarkers during early pregnancy.
A study published in BMC Pregnancy and Childbirth has demonstrated that a combination of fasting plasma glucose (FPG) and insulin-like growth factor binding protein-2 (IGFBP-2) measured between 11 to 14 weeks of gestation can effectively identify women at risk of developing GDM later in their pregnancies.
Gestational diabetes mellitus, characterized by high blood sugar levels that develop during pregnancy, poses significant health risks for both mothers and their infants. Early identification of at-risk women is crucial for implementing preventive measures and improving maternal and fetal outcomes.
It is still uncertain which biomarkers identified in early gestation can effectively predict the later onset of gestational diabetes mellitus. Therefore, Meng-Nan Yang, Xinhua Hospital, Early Life Health Institute, Shanghai Jiao-Tong University School of Medicine, Kong-Jiang Road, Shanghai, China, and colleagues aimed to identify the optimal combination of early gestational biomarkers in predicting GDM in machine learning (ML) models.
For this purpose, the researchers conducted a nested case-control study involving 100 pairs of pregnancies—those with GDM and euglycemic controls—within the Early Life Plan cohort in Shanghai, China. They measured various serum biomarkers at 11-14 weeks of gestation, including high-sensitivity C-reactive protein, sex hormone-binding globulin, insulin-like growth factor I, IGF binding protein-2 (IGFBP-2), total and high molecular weight adiponectin, and glycosylated fibronectin concentrations. Additionally, routine first-trimester blood tests assessed fasting plasma glucose (FPG), serum lipids, and thyroid hormones.
To predict GDM, the researchers employed five machine learning models: stepwise logistic regression, least absolute shrinkage and selection operator (LASSO), random forest, support vector machine, and k-nearest neighbor. The study subjects were randomly divided into two groups: a training set comprising 70 GDM/control pairs and a testing set with 30 pairs. Model performance was evaluated using the area under the curve (AUC) in receiver operating characteristics analysis.
The following were the key findings of the study:
- FPG and IGFBP-2 were consistently selected as predictors of GDM in all ML models.
- The random forest model, including FPG and IGFBP-2, performed the best (AUC 0.80, accuracy 0.72, sensitivity 0.87, specificity 0.57).
- Adding more predictors did not improve the discriminant power.
The researchers acknowledged several limitations in their study, which was conducted within a large pregnancy cohort. Although they developed a machine learning prediction model using only two predictors—where additional clinical features or biomarkers did not improve the predictions—it's important to note that all participants were of Chinese ethnicity. This necessitates further research involving diverse ethnic groups to evaluate the generalizability of the findings.
In conclusion, the combination of fasting plasma glucose and IGFBP-2 during early gestation shows promise in predicting the later development of gestational diabetes mellitus (GDM) with moderate accuracy.
"However, further validation studies are needed to evaluate the effectiveness of this straightforward model in other independent cohorts, as it could serve as a valuable clinical tool for risk monitoring," the researchers wrote.
Reference:
Yang, Meng-Nan, et al. "Prediction of Gestational Diabetes Mellitus By Multiple Biomarkers at Early Gestation." BMC Pregnancy and Childbirth, vol. 24, no. 1, 2024, p. 601.
Dr Kamal Kant Kohli-MBBS, DTCD- a chest specialist with more than 30 years of practice and a flair for writing clinical articles, Dr Kamal Kant Kohli joined Medical Dialogues as a Chief Editor of Medical News. Besides writing articles, as an editor, he proofreads and verifies all the medical content published on Medical Dialogues including those coming from journals, studies,medical conferences,guidelines etc. Email: drkohli@medicaldialogues.in. Contact no. 011-43720751