- Home
- Medical news & Guidelines
- Anesthesiology
- Cardiology and CTVS
- Critical Care
- Dentistry
- Dermatology
- Diabetes and Endocrinology
- ENT
- Gastroenterology
- Medicine
- Nephrology
- Neurology
- Obstretics-Gynaecology
- Oncology
- Ophthalmology
- Orthopaedics
- Pediatrics-Neonatology
- Psychiatry
- Pulmonology
- Radiology
- Surgery
- Urology
- Laboratory Medicine
- Diet
- Nursing
- Paramedical
- Physiotherapy
- Health news
- AYUSH
- State News
- Andaman and Nicobar Islands
- Andhra Pradesh
- Arunachal Pradesh
- Assam
- Bihar
- Chandigarh
- Chattisgarh
- Dadra and Nagar Haveli
- Daman and Diu
- Delhi
- Goa
- Gujarat
- Haryana
- Himachal Pradesh
- Jammu & Kashmir
- Jharkhand
- Karnataka
- Kerala
- Ladakh
- Lakshadweep
- Madhya Pradesh
- Maharashtra
- Manipur
- Meghalaya
- Mizoram
- Nagaland
- Odisha
- Puducherry
- Punjab
- Rajasthan
- Sikkim
- Tamil Nadu
- Telangana
- Tripura
- Uttar Pradesh
- Uttrakhand
- West Bengal
- Medical Education
- Industry
AI-assisted breast-cancer screening may reduce unnecessary testing, finds study
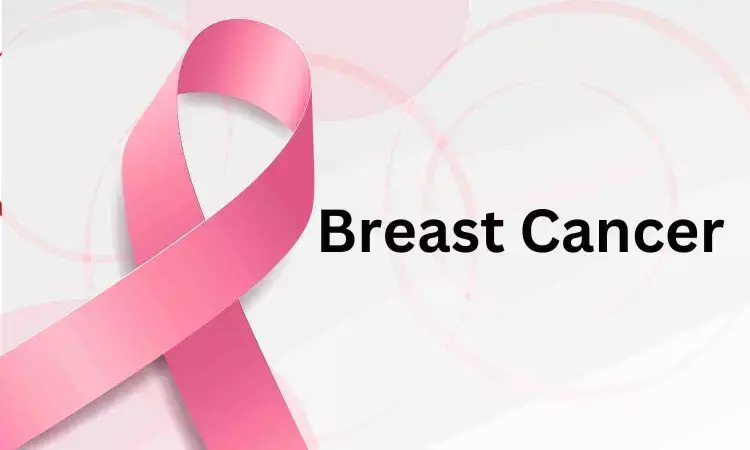
The researchers developed an algorithm that identified normal mammograms with very high sensitivity.They then ran a simulation on patient data to see what would have happened if all of the very low-risk mammograms had been taken off radiologists' plates, freeing the doctors to concentrate on the more questionable scans.The simulation revealed that fewer people would have been called back...
The researchers developed an algorithm that identified normal mammograms with very high sensitivity.
They then ran a simulation on patient data to see what would have happened if all of the very low-risk mammograms had been taken off radiologists' plates, freeing the doctors to concentrate on the more questionable scans.
The simulation revealed that fewer people would have been called back for additional testing but that the same number of cancer cases would have been detected.
"False positives are when you call a patient back for additional testing, and it turns out to be benign," explained senior author Richard L. Wahl, MD, a professor of radiology at Washington University's Mallinckrodt Institute of Radiology (MIR) and a professor of radiation oncology.
"That causes a lot of unnecessary anxiety for patients and consumes medical resources. This simulation study showed that very low-risk mammograms can be reliably identified by AI to reduce false positives and improve workflows."
The study is published April 10 in the journal Radiology: Artificial Intelligence.
Wahl previously collaborated with Whiterabbit.ai on an algorithm to help radiologists judge breast density on mammograms to identify people who could benefit from additional or alternative screening.
That algorithm received clearance from the Food and Drug Administration (FDA) in 2020 and is now marketed by Whiterabbit.ai as WRDensity.
In this study, Wahl and colleagues at Whiterabbit.ai worked together to develop a way to rule out cancer using AI to evaluate mammograms.
They trained the AI model on 123,248 2D digital mammograms (containing 6,161 showing cancer) that were largely collected and read by Washington University radiologists.
Then, they validated and tested the AI model on three independent sets of mammograms, two from institutions in the U.S. and one in the United Kingdom.
First, the researchers figured out what the doctors did: how many patients were called back for secondary screening and biopsies; the results of those tests; and the final determination in each case.
Then, they applied AI to the datasets to see what would have been different if AI had been used to remove negative mammograms in the initial assessments and physicians had followed standard diagnostic procedures to evaluate the rest.
For example, consider the largest dataset, which contained 11,592 mammograms.
When scaled to 10,000 mammograms (to make the math simpler for the purposes of the simulation), AI identified 34.9% as negative.
If those 3,485 negative mammograms had been removed from the workload, radiologists would have made 897 callbacks for diagnostic exams, a reduction of 23.7% from the 1,159 they made in reality.
At the next step, 190 people would have been called in a second time for biopsies, a reduction of 6.9% from the 200 in reality.
At the end of the process, both the AI rule-out and real-world standard-of-care approaches identified the same 55 cancers.
In other words, this study of AI suggests that out of 10,000 people who underwent initial mammograms, 262 could have avoided diagnostic exams, and 10 could have avoided biopsies, without any cancer cases being missed.
"At the end of the day, we believe in a world where the doctor is the superhero who finds cancer and helps patients navigate their journey ahead," said co-author Jason Su, co-founder and chief technology officer at Whiterabbit.ai. "The way AI systems can help is by being in a supporting role. By accurately assessing the negatives, it can help remove the hay from the haystack so doctors can find the needle more easily. This study demonstrates that AI can potentially be highly accurate in identifying negative exams. More importantly, the results showed that automating the detection of negatives may also lead to a tremendous benefit in the reduction of false positives without changing the cancer detection rate."
Reference: Stefano Pedemonte, Trevor Tsue, Brent Mombourquette, Yen Nhi Truong Vu, Thomas Matthews, Rodrigo Morales Hoil, Meet Shah, Nikita Ghare, Naomi Zingman-Daniels, Susan Holley, Catherine M. Appleton, Jason Su, Richard L. Wahl. A Semiautonomous Deep Learning System to Reduce False-Positive Findings in Screening Mammography. Radiology: Artificial Intelligence, 2024; DOI: 10.1148/ryai.230033
Dr Kamal Kant Kohli-MBBS, DTCD- a chest specialist with more than 30 years of practice and a flair for writing clinical articles, Dr Kamal Kant Kohli joined Medical Dialogues as a Chief Editor of Medical News. Besides writing articles, as an editor, he proofreads and verifies all the medical content published on Medical Dialogues including those coming from journals, studies,medical conferences,guidelines etc. Email: editorial@medicaldialogues.in. Contact no. 011-43720751