- Home
- Medical news & Guidelines
- Anesthesiology
- Cardiology and CTVS
- Critical Care
- Dentistry
- Dermatology
- Diabetes and Endocrinology
- ENT
- Gastroenterology
- Medicine
- Nephrology
- Neurology
- Obstretics-Gynaecology
- Oncology
- Ophthalmology
- Orthopaedics
- Pediatrics-Neonatology
- Psychiatry
- Pulmonology
- Radiology
- Surgery
- Urology
- Laboratory Medicine
- Diet
- Nursing
- Paramedical
- Physiotherapy
- Health news
- Fact Check
- Bone Health Fact Check
- Brain Health Fact Check
- Cancer Related Fact Check
- Child Care Fact Check
- Dental and oral health fact check
- Diabetes and metabolic health fact check
- Diet and Nutrition Fact Check
- Eye and ENT Care Fact Check
- Fitness fact check
- Gut health fact check
- Heart health fact check
- Kidney health fact check
- Medical education fact check
- Men's health fact check
- Respiratory fact check
- Skin and hair care fact check
- Vaccine and Immunization fact check
- Women's health fact check
- AYUSH
- State News
- Andaman and Nicobar Islands
- Andhra Pradesh
- Arunachal Pradesh
- Assam
- Bihar
- Chandigarh
- Chattisgarh
- Dadra and Nagar Haveli
- Daman and Diu
- Delhi
- Goa
- Gujarat
- Haryana
- Himachal Pradesh
- Jammu & Kashmir
- Jharkhand
- Karnataka
- Kerala
- Ladakh
- Lakshadweep
- Madhya Pradesh
- Maharashtra
- Manipur
- Meghalaya
- Mizoram
- Nagaland
- Odisha
- Puducherry
- Punjab
- Rajasthan
- Sikkim
- Tamil Nadu
- Telangana
- Tripura
- Uttar Pradesh
- Uttrakhand
- West Bengal
- Medical Education
- Industry
AI based microcalcification detection and classification on mammography may help diagnose breast cancer early
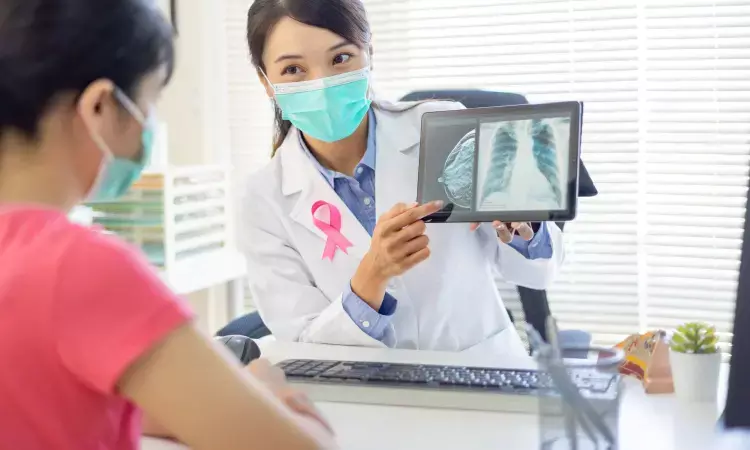
A team led by Lin et al. developed a deep-learning mammography system. This system is trained to identify microcalcification and focuses on interpreting it. Based on the study's findings, a critical perspective is highlighted that using Automated artificial intelligence (AI) systems may assist clinicians in making judgments and improve their efficiency in breast cancer screening, diagnosis, and treatment.
The study Artificial Intelligence-based Diagnosis of breast cancer by mammography microcalcification is published in Fundamental Research.
AI's ability to predict pathological types and ER needs more exploration. Mammography is used for screening of breast cancer screening. Detecting microcalcifications associated with malignancy is vital in diagnosing breast cancer early. Computer-aided diagnosis (CAD) is a promising efficient and accurate technique. In this study, researchers demonstrated an automated deep-learning pipeline for microcalcification detection and classification in mammography.
The team explained, "We built an AI system using deep-learning methods. This includes standardization of images, detection of microcalcification and classification of mammography. Based on Faster RCNN, the model automatically searches for calcification regions in mammography determining benign and malignancy."
FPN structure was used to fuse deep features at different scales as there are small microcalcifications. Considering detection boxes and prediction results presented by the detection model, we determined the category of mammography images (benign or malignant), such as the presence of a malignant box in mammography interpreted as malignant.
The key results of this study are:
- The detection model accurately extracted calcification regions.
- Researchers used a large mammography dataset.
- There were 4,810 mammograms and 6,663 microcalcification lesions.
- The malignant and benign lesions were 3,301 and 3,362, respectively.
- For training and test set, classification accuracy values for discriminating between benign and malignant breasts were 0.8124 and 0.7237, respectively.
- The sensitivity values of malignant breast cancer prediction were 0.8891 and 0.7778, respectively.
- The overall accuracy for the mammograph image level was 0.7055, with an AUC of 0.7753, and 0.7511 for the microcalcification lesion level, with an AUC of 0.7627.
To validate the effectiveness of our deep-learning model, we visualized the feature extraction backbone of our detection network, they said.
They said, "We explored the ability of AI to distinguish between invasive and noninvasive breast cancers and also used it to determine the molecular subtypes of breast carcinoma."
A trained deep-learning model identifying only microcalcification lesions for diagnosing breast cancer early can achieve better accuracy.
We could provide the classification results of mammography images and annotate specific calcification regions. They said this is a rare finding in the literature, and further investigations are warranted in this context.
Further reading:
https://www.sciencedirect.com/science/article/pii/S2667325823001814?via=ihub
BDS, MDS in Periodontics and Implantology
Dr. Aditi Yadav is a BDS, MDS in Periodontics and Implantology. She has a clinical experience of 5 years as a laser dental surgeon. She also has a Diploma in clinical research and pharmacovigilance and is a Certified data scientist. She is currently working as a content developer in e-health services. Dr. Yadav has a keen interest in Medical Journalism and is actively involved in Medical Research writing.
Dr Kamal Kant Kohli-MBBS, DTCD- a chest specialist with more than 30 years of practice and a flair for writing clinical articles, Dr Kamal Kant Kohli joined Medical Dialogues as a Chief Editor of Medical News. Besides writing articles, as an editor, he proofreads and verifies all the medical content published on Medical Dialogues including those coming from journals, studies,medical conferences,guidelines etc. Email: drkohli@medicaldialogues.in. Contact no. 011-43720751