- Home
- Medical news & Guidelines
- Anesthesiology
- Cardiology and CTVS
- Critical Care
- Dentistry
- Dermatology
- Diabetes and Endocrinology
- ENT
- Gastroenterology
- Medicine
- Nephrology
- Neurology
- Obstretics-Gynaecology
- Oncology
- Ophthalmology
- Orthopaedics
- Pediatrics-Neonatology
- Psychiatry
- Pulmonology
- Radiology
- Surgery
- Urology
- Laboratory Medicine
- Diet
- Nursing
- Paramedical
- Physiotherapy
- Health news
- Fact Check
- Bone Health Fact Check
- Brain Health Fact Check
- Cancer Related Fact Check
- Child Care Fact Check
- Dental and oral health fact check
- Diabetes and metabolic health fact check
- Diet and Nutrition Fact Check
- Eye and ENT Care Fact Check
- Fitness fact check
- Gut health fact check
- Heart health fact check
- Kidney health fact check
- Medical education fact check
- Men's health fact check
- Respiratory fact check
- Skin and hair care fact check
- Vaccine and Immunization fact check
- Women's health fact check
- AYUSH
- State News
- Andaman and Nicobar Islands
- Andhra Pradesh
- Arunachal Pradesh
- Assam
- Bihar
- Chandigarh
- Chattisgarh
- Dadra and Nagar Haveli
- Daman and Diu
- Delhi
- Goa
- Gujarat
- Haryana
- Himachal Pradesh
- Jammu & Kashmir
- Jharkhand
- Karnataka
- Kerala
- Ladakh
- Lakshadweep
- Madhya Pradesh
- Maharashtra
- Manipur
- Meghalaya
- Mizoram
- Nagaland
- Odisha
- Puducherry
- Punjab
- Rajasthan
- Sikkim
- Tamil Nadu
- Telangana
- Tripura
- Uttar Pradesh
- Uttrakhand
- West Bengal
- Medical Education
- Industry
AI-powered model may predict prognosis and immunotherapy response in colorectal cancer patients: Study
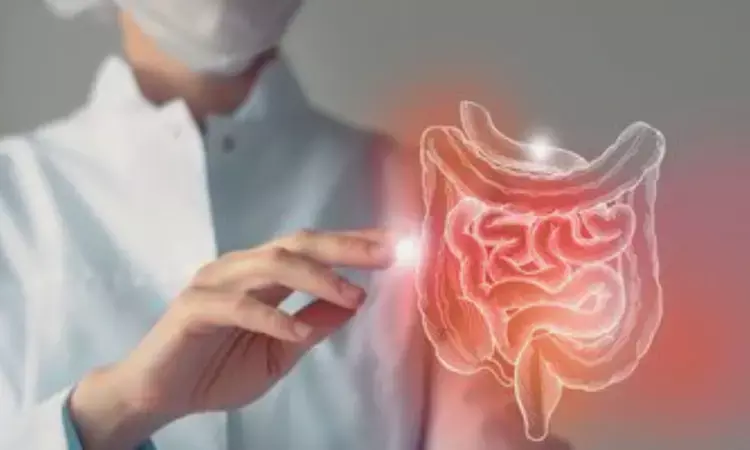
By leveraging multi-omics analysis and machine learning techniques, the research team led by Professor Shuo Wang (Institute of Microbiology, the Chinese Academy of Sciences) developed an Immune Response-related Risk Score (IRRS) model to predict prognosis and immunotherapy response in colorectal cancer (CRC) patients. The study integrated clinical data and transcriptomic profiles from TCGA-CRC and six validation cohorts to identify a robust multi-gene signature.
Through machine learning-based feature selection, the team identified 13 core immune-related genes (IL18BP, RSAD2, G0S2, SIGLEC1, SFRP2, IFI44L, ISG20, IFIT1, OLR1, SAMHD1, HK3, PTAFR, CSF1) that play critical roles in the regulation of the tumor immune microenvironment and response to immunotherapy. The IRRS model, constructed using Random Survival Forest (RSF) and Lasso regression, was validated across multiple datasets and demonstrated superior predictive performance.
In the GSE91061, GSE78220, and IMvigor210 datasets, IRRS effectively stratified patients into high-risk and low-risk groups, with significant survival differences. The ROC analysis confirmed that IRRS outperformed TIDE in predicting immunotherapy response, with higher AUC values across all datasets. Notably, higher IRRS scores correlated with poorer survival and less immune activation, while low IRRS scores were associated with better prognosis and increased immune infiltration.
To further investigate the biological mechanisms underlying IRRS, the researchers conducted immune infiltration analysis and pathway enrichment studies. The results revealed that low-risk IRRS patients exhibited higher levels of immune cell infiltration, particularly CD8+ T cells and natural killer (NK) cells, suggesting an enhanced anti-tumor immune response. Additionally, epigenetic modifications, such as DNA methylation patterns, were analyzed, highlighting potential regulatory mechanisms affecting gene expression in high-risk versus low-risk patients.
“These findings demonstrate that immune-related molecular signatures can serve as reliable predictors of CRC prognosis and immunotherapy response,” said Dr. Wang. “The IRRS model provides a clinically relevant and superior alternative to existing predictive tools, paving the way for more personalized and effective immunotherapy strategies.”
While current scientific consensus suggests that traditional CRC risk models rely heavily on TNM staging, this study presents a paradigm shift by incorporating immune system dynamics into risk assessment. The findings underscore the importance of machine learning and multi-omics data integration in developing precision oncology tools for CRC management.
Future directions include expanding the IRRS model to other cancer types and refining its predictive power through multi-center clinical validation. The study sets the foundation for next-generation precision immunotherapy approaches, encouraging researchers to further explore immune signatures in cancer treatment.
Reference:
Zhen Liu, Dou Yu, Pengyan Xia, Shuo Wang, Machine learning approach to predict prognosis and immunotherapy responses in colorectal cancer patients, hLife, https://doi.org/10.1016/j.hlife.2025.02.001.
Dr Kamal Kant Kohli-MBBS, DTCD- a chest specialist with more than 30 years of practice and a flair for writing clinical articles, Dr Kamal Kant Kohli joined Medical Dialogues as a Chief Editor of Medical News. Besides writing articles, as an editor, he proofreads and verifies all the medical content published on Medical Dialogues including those coming from journals, studies,medical conferences,guidelines etc. Email: drkohli@medicaldialogues.in. Contact no. 011-43720751