- Home
- Medical news & Guidelines
- Anesthesiology
- Cardiology and CTVS
- Critical Care
- Dentistry
- Dermatology
- Diabetes and Endocrinology
- ENT
- Gastroenterology
- Medicine
- Nephrology
- Neurology
- Obstretics-Gynaecology
- Oncology
- Ophthalmology
- Orthopaedics
- Pediatrics-Neonatology
- Psychiatry
- Pulmonology
- Radiology
- Surgery
- Urology
- Laboratory Medicine
- Diet
- Nursing
- Paramedical
- Physiotherapy
- Health news
- Fact Check
- Bone Health Fact Check
- Brain Health Fact Check
- Cancer Related Fact Check
- Child Care Fact Check
- Dental and oral health fact check
- Diabetes and metabolic health fact check
- Diet and Nutrition Fact Check
- Eye and ENT Care Fact Check
- Fitness fact check
- Gut health fact check
- Heart health fact check
- Kidney health fact check
- Medical education fact check
- Men's health fact check
- Respiratory fact check
- Skin and hair care fact check
- Vaccine and Immunization fact check
- Women's health fact check
- AYUSH
- State News
- Andaman and Nicobar Islands
- Andhra Pradesh
- Arunachal Pradesh
- Assam
- Bihar
- Chandigarh
- Chattisgarh
- Dadra and Nagar Haveli
- Daman and Diu
- Delhi
- Goa
- Gujarat
- Haryana
- Himachal Pradesh
- Jammu & Kashmir
- Jharkhand
- Karnataka
- Kerala
- Ladakh
- Lakshadweep
- Madhya Pradesh
- Maharashtra
- Manipur
- Meghalaya
- Mizoram
- Nagaland
- Odisha
- Puducherry
- Punjab
- Rajasthan
- Sikkim
- Tamil Nadu
- Telangana
- Tripura
- Uttar Pradesh
- Uttrakhand
- West Bengal
- Medical Education
- Industry
Machine Learning Model Predicts Schizophrenia More Accurately Than Bipolar Disorder: JAMA
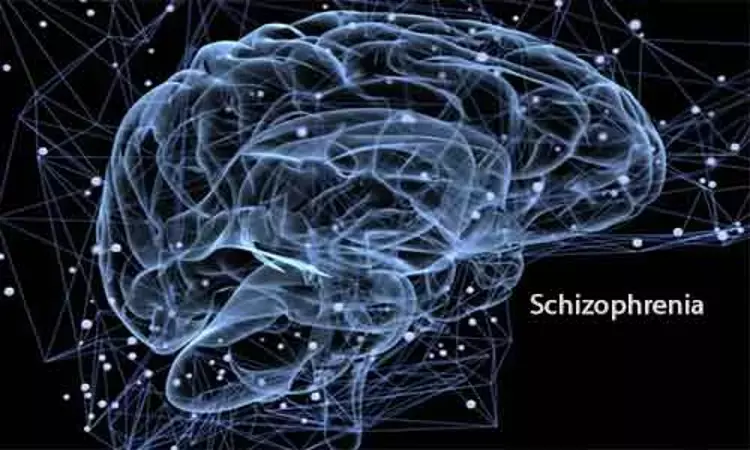
A new study has found that machine learning algorithms can forecast the progression of schizophrenia and bipolar disorder from typical clinical information in electronic health records (EHRs). Schizophrenia and bipolar disorder are typically diagnosed years later when symptoms first occur, although they tend to present during late adolescence or early adulthood. Inconvenient delay in diagnosis can disrupt timely treatment as well as complicate outcomes among patients. This study was conducted by Lasse H. and colleagues published in the journal of JAMA Psychiatry.
The study utilized EHR data from the Central Denmark Region's Psychiatric Services. It involved 24,449 patients aged 15 to 60 with at least two psychiatric service contacts, at least three months between them, between January 1, 2013, and November 21, 2016. The analysis was conducted from December 2022 to November 2024. Predictors were medications, diagnoses, and clinical notes extracted from the EHRs.
To forecast the diagnostic conversion to schizophrenia or bipolar disorder at five years, researchers used two machine learning models: elastic net regularized logistic regression and extreme gradient boosting (XGBoost). The performance of the model was assessed with the area under receiver operating characteristic curve (AUROC).
Key Findings
Conversion to schizophrenia or bipolar disorder was predicted by XGBoost model with an AUROC of 0.70 (95% CI, 0.70-0.70) for the training dataset and 0.64 (95% CI, 0.63-0.65) for the test dataset.
At a predicted positive rate of 4%, the model had:
• Sensitivity: 9.3%
• Specificity: 96.3%
• Positive Predictive Value (PPV): 13.0%
When specifically predicting schizophrenia, the model showed an increased AUROC of 0.80 (95% CI, 0.79-0.81), with:
• Sensitivity: 19.4%
• Specificity: 96.3%
• PPV: 10.8%
• For bipolar disorder, the model was less accurate, with an AUROC of 0.62 (95% CI, 0.61-0.63), sensitivity of 9.9%, specificity of 96.2%, and PPV of 8.4%.
The study authors concluded that machine learning algorithms, specifically XGBoost, were able to predict schizophrenia and bipolar disorder based on EHR information. With increased refinement and testing, AI models could be great assets in psychiatric facilities to help enhance early treatment and diagnosis and ultimately improve patient outcomes.
Reference:
Hansen L, Bernstorff M, Enevoldsen K, et al. Predicting Diagnostic Progression to Schizophrenia or Bipolar Disorder via Machine Learning. JAMA Psychiatry. Published online February 19, 2025. doi:10.1001/jamapsychiatry.2024.4702Dr Riya Dave has completed dentistry from Gujarat University in 2022. She is a dentist and accomplished medical and scientific writer known for her commitment to bridging the gap between clinical expertise and accessible healthcare information. She has been actively involved in writing blogs related to health and wellness.
Dr Kamal Kant Kohli-MBBS, DTCD- a chest specialist with more than 30 years of practice and a flair for writing clinical articles, Dr Kamal Kant Kohli joined Medical Dialogues as a Chief Editor of Medical News. Besides writing articles, as an editor, he proofreads and verifies all the medical content published on Medical Dialogues including those coming from journals, studies,medical conferences,guidelines etc. Email: drkohli@medicaldialogues.in. Contact no. 011-43720751