- Home
- Medical news & Guidelines
- Anesthesiology
- Cardiology and CTVS
- Critical Care
- Dentistry
- Dermatology
- Diabetes and Endocrinology
- ENT
- Gastroenterology
- Medicine
- Nephrology
- Neurology
- Obstretics-Gynaecology
- Oncology
- Ophthalmology
- Orthopaedics
- Pediatrics-Neonatology
- Psychiatry
- Pulmonology
- Radiology
- Surgery
- Urology
- Laboratory Medicine
- Diet
- Nursing
- Paramedical
- Physiotherapy
- Health news
- Fact Check
- Bone Health Fact Check
- Brain Health Fact Check
- Cancer Related Fact Check
- Child Care Fact Check
- Dental and oral health fact check
- Diabetes and metabolic health fact check
- Diet and Nutrition Fact Check
- Eye and ENT Care Fact Check
- Fitness fact check
- Gut health fact check
- Heart health fact check
- Kidney health fact check
- Medical education fact check
- Men's health fact check
- Respiratory fact check
- Skin and hair care fact check
- Vaccine and Immunization fact check
- Women's health fact check
- AYUSH
- State News
- Andaman and Nicobar Islands
- Andhra Pradesh
- Arunachal Pradesh
- Assam
- Bihar
- Chandigarh
- Chattisgarh
- Dadra and Nagar Haveli
- Daman and Diu
- Delhi
- Goa
- Gujarat
- Haryana
- Himachal Pradesh
- Jammu & Kashmir
- Jharkhand
- Karnataka
- Kerala
- Ladakh
- Lakshadweep
- Madhya Pradesh
- Maharashtra
- Manipur
- Meghalaya
- Mizoram
- Nagaland
- Odisha
- Puducherry
- Punjab
- Rajasthan
- Sikkim
- Tamil Nadu
- Telangana
- Tripura
- Uttar Pradesh
- Uttrakhand
- West Bengal
- Medical Education
- Industry
AI model may improve pulmonary nodule detection on chest radiograph: JAMA
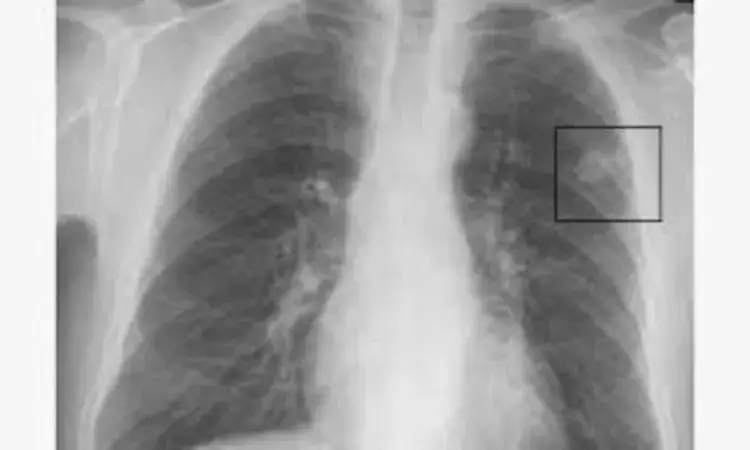
Korea: A group of researchers from Korea has developed an AI (artificial intelligence) model that could help radiologists with pulmonary nodule detection on chest radiographs.
The decision analytical modeling study, published in JAMA Network Open, found that DLBS (deep learning–based synthetic bone-suppressed) model was more sensitive to pulmonary nodule detection on chest radiographs than the original model. The model helped radiologists reach sensitivities of 92% for detecting lung nodules.
Dual-energy chest radiography is a technique for improving diagnostic accuracy in chest imaging by separating soft tissues from bones during a single x-ray exposure, thus enhancing the visualization of underlying and overlying anatomy. The technique, however, requires increasing radiation exposure to patients and specialized equipment.
In their previous work, Hwiyoung Kim from Yonsei University College of Medicine in Seoul in, Korea, and colleagues developed a deep-learning model to identify high-quality bone- and soft tissue-only images through standard equipment. In the study, the team aimed to further improve the model by adding a bone-suppression algorithm and then the radiologists' tested its use.
The researchers used data from 3 centres between 2015 and 2019 from 1449 patients. The DLBS nodule-detection algorithm was trained using single-centre data from 998 chest radiographs (institute 1). Validation was done using two external data sets (institute 3, 205 patients; and institute 2, 246 patients).
A comparison was made between the nodule-detection performance of the DLBS model and the convolution neural network nodule-detection algorithm (original model). Three thoracic radiologists assisted by the DLBS algorithm or not tested the performance testing. False-positive markings per image (FPPI) and sensitivity were compared.
The study led to the following findings:
- Training data consisted of 998 patients (54.0% were men; mean age, 54.2 years), and two external validation data sets consisted of 246 patients (54.1% were men; mean age, 55.3 years) and 205 patients (151.2% were men; mean age, 51.8 years).
- The bone-suppressed model revealed higher sensitivity than the original model for nodule detection (91.5% vs 79.8%) using the external validation data set of institute 2.
- There was a reduction in the overall mean of FPPI with the bone-suppressed model compared with the original model (0.07 vs 0.09).
- For the observer performance testing with the data of institute 3, the mean sensitivity of 3 radiologists was 77.5%, whereas that of radiologists assisted by DLBS modeling was 92.1%.
- When assisted by the DLBS model, the three radiologists had a reduced number of FPPI (0.071 vs 0.151).
"Our findings indicate that the DLBS model could benefit radiologists in the lung nodules detection in chest radiographs without the need for specialized equipment or an increase of radiation dose," the team concluded.
Reference:
Kim H, Lee KH, Han K, et al. Development and Validation of a Deep Learning–Based Synthetic Bone-Suppressed Model for Pulmonary Nodule Detection in Chest Radiographs. JAMA Netw Open. 2023;6(1):e2253820. doi:10.1001/jamanetworkopen.2022.53820
MSc. Biotechnology
Medha Baranwal joined Medical Dialogues as an Editor in 2018 for Speciality Medical Dialogues. She covers several medical specialties including Cardiac Sciences, Dentistry, Diabetes and Endo, Diagnostics, ENT, Gastroenterology, Neurosciences, and Radiology. She has completed her Bachelors in Biomedical Sciences from DU and then pursued Masters in Biotechnology from Amity University. She has a working experience of 5 years in the field of medical research writing, scientific writing, content writing, and content management. She can be contacted at  editorial@medicaldialogues.in. Contact no. 011-43720751
Dr Kamal Kant Kohli-MBBS, DTCD- a chest specialist with more than 30 years of practice and a flair for writing clinical articles, Dr Kamal Kant Kohli joined Medical Dialogues as a Chief Editor of Medical News. Besides writing articles, as an editor, he proofreads and verifies all the medical content published on Medical Dialogues including those coming from journals, studies,medical conferences,guidelines etc. Email: drkohli@medicaldialogues.in. Contact no. 011-43720751