- Home
- Medical news & Guidelines
- Anesthesiology
- Cardiology and CTVS
- Critical Care
- Dentistry
- Dermatology
- Diabetes and Endocrinology
- ENT
- Gastroenterology
- Medicine
- Nephrology
- Neurology
- Obstretics-Gynaecology
- Oncology
- Ophthalmology
- Orthopaedics
- Pediatrics-Neonatology
- Psychiatry
- Pulmonology
- Radiology
- Surgery
- Urology
- Laboratory Medicine
- Diet
- Nursing
- Paramedical
- Physiotherapy
- Health news
- Fact Check
- Bone Health Fact Check
- Brain Health Fact Check
- Cancer Related Fact Check
- Child Care Fact Check
- Dental and oral health fact check
- Diabetes and metabolic health fact check
- Diet and Nutrition Fact Check
- Eye and ENT Care Fact Check
- Fitness fact check
- Gut health fact check
- Heart health fact check
- Kidney health fact check
- Medical education fact check
- Men's health fact check
- Respiratory fact check
- Skin and hair care fact check
- Vaccine and Immunization fact check
- Women's health fact check
- AYUSH
- State News
- Andaman and Nicobar Islands
- Andhra Pradesh
- Arunachal Pradesh
- Assam
- Bihar
- Chandigarh
- Chattisgarh
- Dadra and Nagar Haveli
- Daman and Diu
- Delhi
- Goa
- Gujarat
- Haryana
- Himachal Pradesh
- Jammu & Kashmir
- Jharkhand
- Karnataka
- Kerala
- Ladakh
- Lakshadweep
- Madhya Pradesh
- Maharashtra
- Manipur
- Meghalaya
- Mizoram
- Nagaland
- Odisha
- Puducherry
- Punjab
- Rajasthan
- Sikkim
- Tamil Nadu
- Telangana
- Tripura
- Uttar Pradesh
- Uttrakhand
- West Bengal
- Medical Education
- Industry
3D structural brain MRI using deep learning exhibits potential for detection of schizophrenia
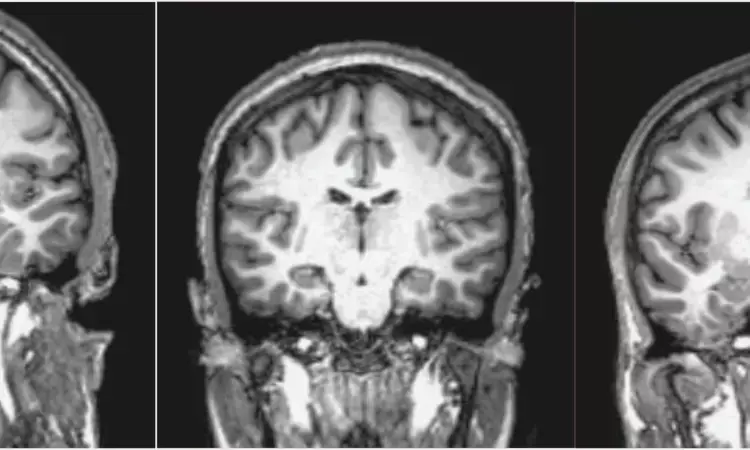
USA: A recent study published in Scientific Reports has shown the potential of deep learning for improving the diagnosis of schizophrenia using a single, standard T1-weighted brain MRI.deep learning
Schizophrenia is a chronic neuropsychiatric disorder that leads to distinct structural alterations within the brain. Jia Guo, Department of Psychiatry, Columbia University, New York, NY, USA, and colleagues hypothesized that deep learning applied to a structural neuroimaging dataset could detect disease-related alteration and improve classification and diagnostic accuracy.
They tested this hypothesis using a widely available, single, and conventional T1-weighted MRI scan, from which a 3D whole-brain structure was extracted using standard post-processing methods. Then, a deep-learning model was developed, optimized, and evaluated on three open datasets with T1-weighted MRI scans of schizophrenia.
For the study, Dr. Guo and the team implemented the schizophrenia classification task with the benchmark model using 887 structural whole-head (WH) T1W scans, following the same pre-processing and parameter setting as CNN benchmark implementation. They used a modified 3D VGG18 with squeeze excitation (SE)19 and batch normalization (BN)20 model (SE-VGG-11BN) to perform the schizophrenia versus cognitive normal binary classification task with the input of 887 T1W structural whole-brain (WB) scans. 437 were controls and 450 were schizophrenia patients from the 887 scans.
The proposed model showcased superior generality and performance compared to the benchmark model in terms of accuracy, specificity, and AUC in both experiments. Additionally, the proposed model, SE-VGG11-BN, was interpreted with gradient class activation maps for the visualization of brain regions critical for classification. The important regions for classification involved ventricular and subcortical areas; these were in line with the findings in the previous literature.
The investigators showed that the model outperformed the benchmark model, which was also trained with structural MR images using a 3D CNN architecture.
"Our model had the potential to almost perfectly (area under the ROC curve = 0.987) distinguish schizophrenia patients from healthy controls on unseen structural MRI scans," the researchers wrote.
They noted that regional analysis localized subcortical regions and ventricles as the most predictive brain regions. Subcortical structures serve an important role in affective, cognitive, and social functions in humans, and structural abnormalities of these regions have been associated with schizophrenia.
The findings of the study corroborate that schizophrenia is linked with widespread alterations in subcortical brain structure and the subcortical structural information provides prominent features in diagnostic classification.
"Together, these findings further demonstrate the potential of deep learning to improve schizophrenia diagnosis and identify its structural neuroimaging signatures from a single, standard T1-weighted brain MRI," the researchers concluded.
Reference:
Zhang, J., Rao, V. M., Tian, Y., Yang, Y., Acosta, N., Wan, Z., Lee, P., Zhang, C., Kegeles, L. S., Small, S. A., & Guo, J. (2023). Detecting schizophrenia with 3D structural brain MRI using deep learning. Scientific Reports, 13(1), 1-10. https://doi.org/10.1038/s41598-023-41359-z
Dr Kamal Kant Kohli-MBBS, DTCD- a chest specialist with more than 30 years of practice and a flair for writing clinical articles, Dr Kamal Kant Kohli joined Medical Dialogues as a Chief Editor of Medical News. Besides writing articles, as an editor, he proofreads and verifies all the medical content published on Medical Dialogues including those coming from journals, studies,medical conferences,guidelines etc. Email: drkohli@medicaldialogues.in. Contact no. 011-43720751