- Home
- Medical news & Guidelines
- Anesthesiology
- Cardiology and CTVS
- Critical Care
- Dentistry
- Dermatology
- Diabetes and Endocrinology
- ENT
- Gastroenterology
- Medicine
- Nephrology
- Neurology
- Obstretics-Gynaecology
- Oncology
- Ophthalmology
- Orthopaedics
- Pediatrics-Neonatology
- Psychiatry
- Pulmonology
- Radiology
- Surgery
- Urology
- Laboratory Medicine
- Diet
- Nursing
- Paramedical
- Physiotherapy
- Health news
- Fact Check
- Bone Health Fact Check
- Brain Health Fact Check
- Cancer Related Fact Check
- Child Care Fact Check
- Dental and oral health fact check
- Diabetes and metabolic health fact check
- Diet and Nutrition Fact Check
- Eye and ENT Care Fact Check
- Fitness fact check
- Gut health fact check
- Heart health fact check
- Kidney health fact check
- Medical education fact check
- Men's health fact check
- Respiratory fact check
- Skin and hair care fact check
- Vaccine and Immunization fact check
- Women's health fact check
- AYUSH
- State News
- Andaman and Nicobar Islands
- Andhra Pradesh
- Arunachal Pradesh
- Assam
- Bihar
- Chandigarh
- Chattisgarh
- Dadra and Nagar Haveli
- Daman and Diu
- Delhi
- Goa
- Gujarat
- Haryana
- Himachal Pradesh
- Jammu & Kashmir
- Jharkhand
- Karnataka
- Kerala
- Ladakh
- Lakshadweep
- Madhya Pradesh
- Maharashtra
- Manipur
- Meghalaya
- Mizoram
- Nagaland
- Odisha
- Puducherry
- Punjab
- Rajasthan
- Sikkim
- Tamil Nadu
- Telangana
- Tripura
- Uttar Pradesh
- Uttrakhand
- West Bengal
- Medical Education
- Industry
AI algorithm improves fracture diagnosis on whole-body trauma computed tomography: Study
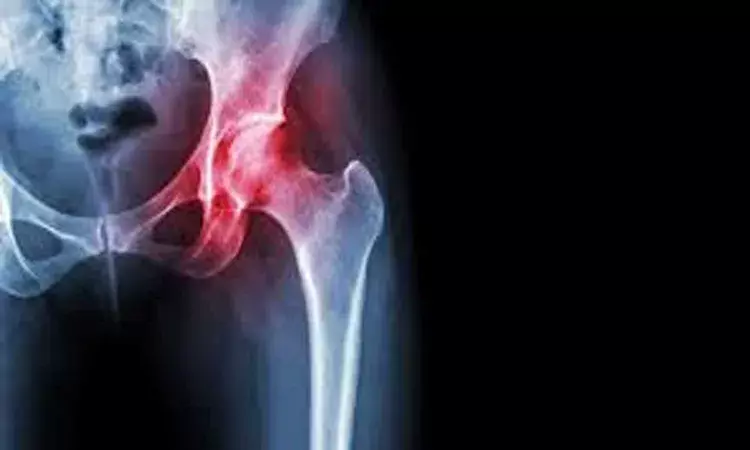
Japan: An artificial intelligence (AI) algorithm can improve fracture detection on whole-body trauma computed tomography (CT) exams performed on patients in emergency departments, researchers state in a recent study published in Scientific Reports.
Following training in a convolutional neural network (CNN), the researchers found that it remarkably increased the sensitivity of orthopedic surgeons for detecting rib, pelvic, and spine fractures. CNN model application may reduce missed fractures from whole-body CT images, lead to faster workflows, and improve patient care through efficient diagnosis in polytrauma patients.
The emergency department is an environment with a possible risk for diagnostic errors during trauma care, specifically for fractures. CNN deep learning methods are used widely in medicine as they improve diagnostic accuracy and efficiency and decrease misinterpretation. The research team investigated whether automatic localization and classification utilizing CNN could be applied to the spine, rib, and pelvic fractures. They also examined if this fracture detection algorithm could be helpful for physicians in diagnosing fractures.
For this purpose, Satoshi Maki, Center for Frontier Medical Engineering, Chiba University, Chiba, Japan, and colleagues used a total of 7664 whole-body CT axial slices (pelvis, abdomen, chest) from 200 patients. The calculation of precision, sensitivity, and F1-score were done to evaluate the CNN model's performance.
The study's key findings were as follows:
- For the grouped mean values for pelvic, spine, or rib fractures, the sensitivity was 0.786, precision was 0.648, and F1-score was 0.711.
- With the assistance of the CNN model, surgeons showed improved sensitivity for detecting fractures, and the time of reading and interpreting CT scans was reduced, especially for less experienced orthopedic surgeons.
- AI also sharply decreased the time to diagnosis, dropping from 278.4 seconds to 162.3 seconds for surgeon 1, from 205.2 seconds to 144.5 seconds for surgeon 2, and from 233.7 seconds to 155.5 seconds for surgeon 3. All differences were statistically significant.
"CNN model application reduced missed fractures from whole-body CT images, led to faster workflows, and improves patient care through efficient diagnosis in polytrauma patients," the researchers wrote in their conclusion.
They noted that CNN could serve as a triage system in a busy emergency department.
"If CNN can diagnose fracture before orthopedic surgeons or radiologists have a prospect for image reviewal, the suspected fracture could become a high priority in the worklist," the researchers wrote. "If the interpretation of images can be prioritized with potentially positive findings, diagnosis delays can be minimized, therefore improving patient care."
Reference:
Inoue, T., Maki, S., Furuya, T. et al. Automated fracture screening using an object detection algorithm on whole-body trauma computed tomography. Sci Rep 12, 16549 (2022). https://doi.org/10.1038/s41598-022-20996-w
MSc. Biotechnology
Medha Baranwal joined Medical Dialogues as an Editor in 2018 for Speciality Medical Dialogues. She covers several medical specialties including Cardiac Sciences, Dentistry, Diabetes and Endo, Diagnostics, ENT, Gastroenterology, Neurosciences, and Radiology. She has completed her Bachelors in Biomedical Sciences from DU and then pursued Masters in Biotechnology from Amity University. She has a working experience of 5 years in the field of medical research writing, scientific writing, content writing, and content management. She can be contacted at  editorial@medicaldialogues.in. Contact no. 011-43720751
Dr Kamal Kant Kohli-MBBS, DTCD- a chest specialist with more than 30 years of practice and a flair for writing clinical articles, Dr Kamal Kant Kohli joined Medical Dialogues as a Chief Editor of Medical News. Besides writing articles, as an editor, he proofreads and verifies all the medical content published on Medical Dialogues including those coming from journals, studies,medical conferences,guidelines etc. Email: drkohli@medicaldialogues.in. Contact no. 011-43720751