- Home
- Medical news & Guidelines
- Anesthesiology
- Cardiology and CTVS
- Critical Care
- Dentistry
- Dermatology
- Diabetes and Endocrinology
- ENT
- Gastroenterology
- Medicine
- Nephrology
- Neurology
- Obstretics-Gynaecology
- Oncology
- Ophthalmology
- Orthopaedics
- Pediatrics-Neonatology
- Psychiatry
- Pulmonology
- Radiology
- Surgery
- Urology
- Laboratory Medicine
- Diet
- Nursing
- Paramedical
- Physiotherapy
- Health news
- Fact Check
- Bone Health Fact Check
- Brain Health Fact Check
- Cancer Related Fact Check
- Child Care Fact Check
- Dental and oral health fact check
- Diabetes and metabolic health fact check
- Diet and Nutrition Fact Check
- Eye and ENT Care Fact Check
- Fitness fact check
- Gut health fact check
- Heart health fact check
- Kidney health fact check
- Medical education fact check
- Men's health fact check
- Respiratory fact check
- Skin and hair care fact check
- Vaccine and Immunization fact check
- Women's health fact check
- AYUSH
- State News
- Andaman and Nicobar Islands
- Andhra Pradesh
- Arunachal Pradesh
- Assam
- Bihar
- Chandigarh
- Chattisgarh
- Dadra and Nagar Haveli
- Daman and Diu
- Delhi
- Goa
- Gujarat
- Haryana
- Himachal Pradesh
- Jammu & Kashmir
- Jharkhand
- Karnataka
- Kerala
- Ladakh
- Lakshadweep
- Madhya Pradesh
- Maharashtra
- Manipur
- Meghalaya
- Mizoram
- Nagaland
- Odisha
- Puducherry
- Punjab
- Rajasthan
- Sikkim
- Tamil Nadu
- Telangana
- Tripura
- Uttar Pradesh
- Uttrakhand
- West Bengal
- Medical Education
- Industry
Automated deep learning AI tool with CT imaging facilitates screening for enlarged spleen: Study
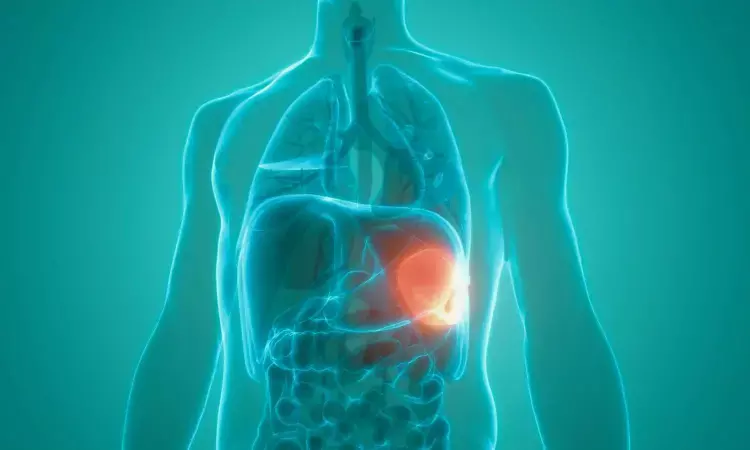
USA: A research team from the US has derived a weight-based volumetric threshold for splenomegaly (spleen enlargement) using an automated AI-based tool.
In their study published in the American Journal of Roentgenology, the researchers revealed that using a deep-learning AI (artificial intelligence) algorithm and weight-based volumetric thresholds with CT (computed tomography) imaging is promising in screening for an enlarged spleen.
"The findings are relevant for patients, particularly those with end-stage liver disease and those who are about to undergo a liver transplant," Alberto A. Perez, Mallinckrodt Institute of Radiology, St. Louis, MO, and colleagues wrote in their study.
Splenomegaly has historically been evaluated on imaging using potentially inaccurate linear measurements. Prior studies tested a deep-learning AI tool that automatically segments the spleen to determine splenic volume. Therefore, Dr Perez and the team aimed to apply the deep-learning AI tool in a large screening population to establish volume-based splenomegaly thresholds using a retrospective study.
The study included a primary sample comprising 8901 patients (mean age, 56±10 years) who underwent CT colonoscopy (n=7736) or renal-donor CT (n=1165) from 2004 to 2017. A secondary sample comprised 104 patients (mean age 56±8 years) with ESLD (end-stage liver disease) who underwent pre-liver transplant CT from 2011 to 2013.
The automated deep-learning AI tool was used to determine splenic volumes for spleen segmentation. Two radiologists reviewed a subset of segmentations. A regression analysis was used to derive weight-based volume thresholds for splenomegaly. The authors evaluated the performance of linear measurements. The frequency of splenomegaly was determined using weight-based volumetric thresholds in the second sample.
The authors reported the following findings:
- In the primary sample, both observers confirmed splenectomy in 20 patients with an automated splenic volume of zero; confirmed incomplete splenic coverage in 28 patients with a tool output error; and confirmed adequate segmentation in 21 patients with low (<50 cc) volume, 49 patients with high (>600 cc) volume, and 200 additional randomly selected patients.
- In 8853 patients included in the analysis of splenic volumes (i.e., excluding zero or error values), the mean automated splenic volume was 216±100 ml.
- Weight-based volumetric threshold (in millilitres) for splenomegaly was defined as 3.0*(weight[kg])+127; for weight >125 kg, the splenomegaly threshold was constant (503 ml).
- Sensitivity and specificity for volume-defined splenomegaly were 13% and 100% at a true-craniocaudal length of 13 cm and 78% and 88% for a maximum 3D length of 13 cm.
- In the secondary sample, both observers identified segmentation failure in one patient.
- The mean automated splenic volume in the 103 remaining patients was 796±457 ml; 84% met the weight-based volume-defined splenomegaly threshold.
Based on the findings, the adequate performance of the deep-learning algorithm for splenic volume evaluation could be an essential additional tool for patient assessment.
"Use of the automated deep-learning AI tool and weight-based volumetric thresholds could allow large-scale evaluation for splenomegaly on CT examinations performed for any indication," they concluded.
Reference:
Perez AA, Noe-Kim V, Lubner MG, Somsen D, Garrett JW, Summers RM, Pickhardt PJ. Automated Deep Learning Artificial Intelligence Tool for Spleen Segmentation on CT: Defining Volume-Based Thresholds for Splenomegaly. AJR Am J Roentgenol. 2023 Jun 28. doi: 10.2214/AJR.23.29478. Epub ahead of print. PMID: 37377359.
MSc. Biotechnology
Medha Baranwal joined Medical Dialogues as an Editor in 2018 for Speciality Medical Dialogues. She covers several medical specialties including Cardiac Sciences, Dentistry, Diabetes and Endo, Diagnostics, ENT, Gastroenterology, Neurosciences, and Radiology. She has completed her Bachelors in Biomedical Sciences from DU and then pursued Masters in Biotechnology from Amity University. She has a working experience of 5 years in the field of medical research writing, scientific writing, content writing, and content management. She can be contacted at  editorial@medicaldialogues.in. Contact no. 011-43720751
Dr Kamal Kant Kohli-MBBS, DTCD- a chest specialist with more than 30 years of practice and a flair for writing clinical articles, Dr Kamal Kant Kohli joined Medical Dialogues as a Chief Editor of Medical News. Besides writing articles, as an editor, he proofreads and verifies all the medical content published on Medical Dialogues including those coming from journals, studies,medical conferences,guidelines etc. Email: drkohli@medicaldialogues.in. Contact no. 011-43720751