- Home
- Medical news & Guidelines
- Anesthesiology
- Cardiology and CTVS
- Critical Care
- Dentistry
- Dermatology
- Diabetes and Endocrinology
- ENT
- Gastroenterology
- Medicine
- Nephrology
- Neurology
- Obstretics-Gynaecology
- Oncology
- Ophthalmology
- Orthopaedics
- Pediatrics-Neonatology
- Psychiatry
- Pulmonology
- Radiology
- Surgery
- Urology
- Laboratory Medicine
- Diet
- Nursing
- Paramedical
- Physiotherapy
- Health news
- Fact Check
- Bone Health Fact Check
- Brain Health Fact Check
- Cancer Related Fact Check
- Child Care Fact Check
- Dental and oral health fact check
- Diabetes and metabolic health fact check
- Diet and Nutrition Fact Check
- Eye and ENT Care Fact Check
- Fitness fact check
- Gut health fact check
- Heart health fact check
- Kidney health fact check
- Medical education fact check
- Men's health fact check
- Respiratory fact check
- Skin and hair care fact check
- Vaccine and Immunization fact check
- Women's health fact check
- AYUSH
- State News
- Andaman and Nicobar Islands
- Andhra Pradesh
- Arunachal Pradesh
- Assam
- Bihar
- Chandigarh
- Chattisgarh
- Dadra and Nagar Haveli
- Daman and Diu
- Delhi
- Goa
- Gujarat
- Haryana
- Himachal Pradesh
- Jammu & Kashmir
- Jharkhand
- Karnataka
- Kerala
- Ladakh
- Lakshadweep
- Madhya Pradesh
- Maharashtra
- Manipur
- Meghalaya
- Mizoram
- Nagaland
- Odisha
- Puducherry
- Punjab
- Rajasthan
- Sikkim
- Tamil Nadu
- Telangana
- Tripura
- Uttar Pradesh
- Uttrakhand
- West Bengal
- Medical Education
- Industry
New AI Model Improves Preoperative Severity Prediction for Pediatric Appendicitis: Study
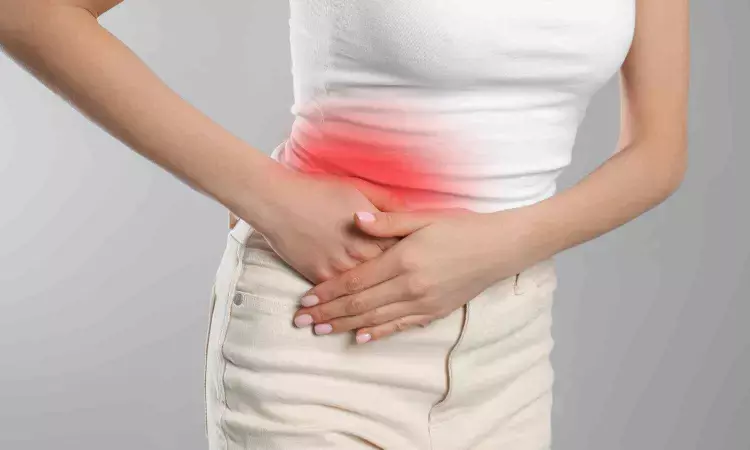
Canada: A new machine-learning model has demonstrated potential in predicting the severity of pediatric appendicitis before surgery.
"The model successfully classified appendicitis into five severity grades, achieving an accuracy of 70.1% and an AUROC of 0.77. It also effectively distinguished between non-perforated and perforated appendicitis, with a negative predictive value (NPV) of 82.8% and a positive predictive value (PPV) of 56.4%," the researchers wrote in the Journal of Pediatric Surgery.
Aylin Erman, Department of Computer Science, McGill University, Montreal, QC, and colleagues aimed to assess the effectiveness of machine learning (ML) algorithms in enhancing the preoperative diagnosis of acute appendicitis in children, with an emphasis on accurately predicting disease severity.
For this purpose, the researchers retrieved an anonymized clinical and operative dataset from the medical records of children who underwent emergency appendectomy between 2014 and 2021. They developed a machine learning (ML) pipeline to preprocess the dataset and create algorithms that predict five appendicitis severity grades: 1 - non-perforated, 2 - localized perforation, 3 - abscess, 4 - generalized peritonitis, and 5 - generalized peritonitis with abscess.
Missing values were imputed, and infrequent classes were addressed using upsampling techniques. Various classifier models were tested, with the best combination of imputation strategy, class balancing, and classification model selected based on validation performance. The model’s explainability was confirmed by a pediatric surgeon and compared with another pediatric appendicitis severity prediction tool.
The study led to the following findings:
- The study analyzed a retrospective cohort of 1,980 patients (60.6% male, average age 10.7 years).
- The distribution of appendicitis severity grades in the cohort was: grade 1 (70%), grade 2 (8%), grade 3 (7%), grade 4 (7%), and grade 5 (8%).
- The researchers tested every combination of 6 imputation strategies, seven class-balancing techniques, and five classification models.
- The best-performing machine learning pipeline achieved:
- 82.8% NPV and 56.4% PPV in distinguishing non-perforated from perforated appendicitis.
- 70.1% accuracy and an AUROC of 0.77 in differentiating between severity grades.
- A comparison with another pediatric appendicitis severity prediction tool showed:
- 71.4% accuracy, AUROC of 0.54, and NPV/PPV of 71.8%/64.7%.
In conclusion, the study demonstrates that the machine learning model for predicting appendiceal perforation outperforms models predicting the full spectrum of appendicitis grades. The key variables identified by the model align with clinical experience and existing literature, indicating that the ML approach successfully identified relevant patterns in the data. Notably, this model surpasses other pediatric appendicitis prediction tools in performance.
"Our ML model for grade prediction represents a novel method for assessing appendicitis severity in children preoperatively. Following further validation and clinical testing, it has the potential to enable personalized treatment and optimize resource allocation in managing pediatric appendicitis," the researchers concluded.
Reference:
Erman, A., Ferreira, J., Ashour, W. A., Guadagno, E., St-Louis, E., Emil, S., Cheung, J., & Poenaru, D. (2025). Machine-learning-assisted preoperative prediction of pediatric appendicitis severity. Journal of Pediatric Surgery, 162151. https://doi.org/10.1016/j.jpedsurg.2024.162151
MSc. Biotechnology
Medha Baranwal joined Medical Dialogues as an Editor in 2018 for Speciality Medical Dialogues. She covers several medical specialties including Cardiac Sciences, Dentistry, Diabetes and Endo, Diagnostics, ENT, Gastroenterology, Neurosciences, and Radiology. She has completed her Bachelors in Biomedical Sciences from DU and then pursued Masters in Biotechnology from Amity University. She has a working experience of 5 years in the field of medical research writing, scientific writing, content writing, and content management. She can be contacted at  editorial@medicaldialogues.in. Contact no. 011-43720751
Dr Kamal Kant Kohli-MBBS, DTCD- a chest specialist with more than 30 years of practice and a flair for writing clinical articles, Dr Kamal Kant Kohli joined Medical Dialogues as a Chief Editor of Medical News. Besides writing articles, as an editor, he proofreads and verifies all the medical content published on Medical Dialogues including those coming from journals, studies,medical conferences,guidelines etc. Email: drkohli@medicaldialogues.in. Contact no. 011-43720751