Automated Machine Learning Classifier valuable for Early Childhood Caries: Study
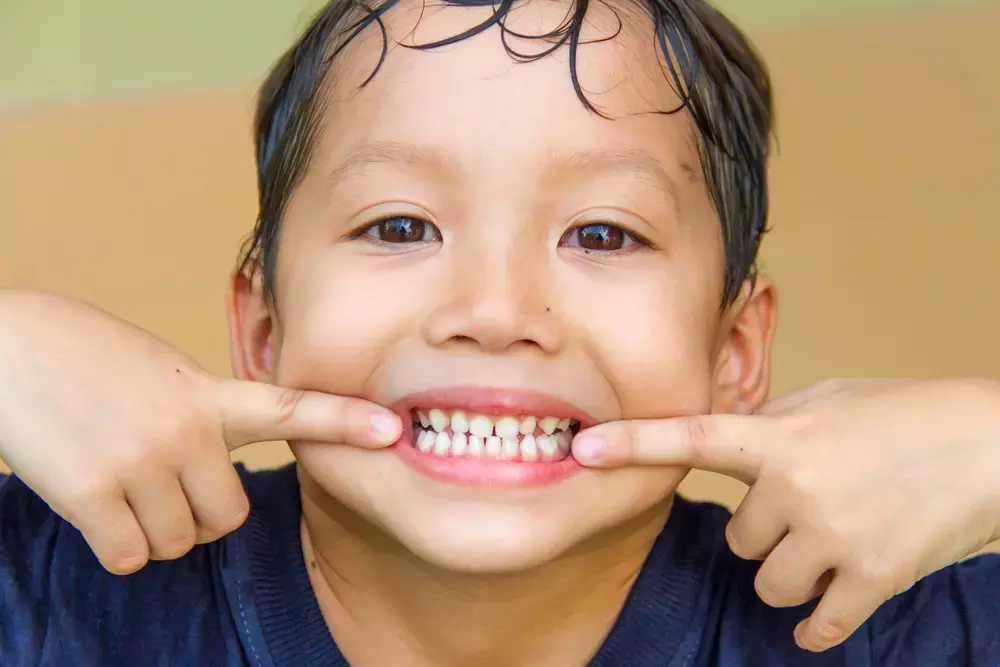
An Automated Machine Learning Classifier for Early Childhood Caries can be valuable, according to a study published in the American Academy of Pediatric Dentistry.
A group of researchers from the U.S.A conducted a study to develop and evaluate an automated machine learning algorithm (AutoML) for children's classification according to early childhood caries (ECC) status.
Clinical, demographic, behavioural, and parent-reported oral health status information for a sample of 6,404 three- to five-year-old children (mean age equals 54 months) participating in an epidemiologic study of early childhood oral health in North Carolina was used.
Early childhood caries (ECC) prevalence (decayed, missing, and filled primary teeth surfaces [dmfs] score greater than zero, using an International Caries Detection and Assessment System score greater than or equal to three caries lesion detection threshold) was 54 percent. Ten sets of ECC predictors were evaluated for ECC classification accuracy (i.e., area under the ROC curve [AUC], sensitivity [Se], and positive predictive value [PPV]) using an AutoML deployment on Google Cloud, followed by internal validation and external replication.
The results of the study are as follows:
A parsimonious model including two terms (i.e., children's age and parent-reported child oral health status: excellent/very good/good/fair/poor) had the highest AUC (0.74), Se (0.67), and PPV (0.64) scores and similar performance using an external National Health and Nutrition Examination Survey (NHANES) dataset (AUC equals 0.80, Se equals 0.73, PPV equals 0.49). Contrarily, a comprehensive model with 12 variables covering demographics (e.g., race/ethnicity, parental education), oral health behaviours, fluoride exposure, and dental home had worse performance.
Thus, the researchers concluded that parsimonious automated machine learning early childhood caries classifiers, including single-item self-reports, can be valuable for Early Childhood Caries screening. The classifier can accommodate biological information that can help improve its performance in the future.
Reference:
An Automated Machine Learning Classifier for Early Childhood Caries by Karhade D et. al published in the American Academy of Pediatric Dentistry
Disclaimer: This website is primarily for healthcare professionals. The content here does not replace medical advice and should not be used as medical, diagnostic, endorsement, treatment, or prescription advice. Medical science evolves rapidly, and we strive to keep our information current. If you find any discrepancies, please contact us at corrections@medicaldialogues.in. Read our Correction Policy here. Nothing here should be used as a substitute for medical advice, diagnosis, or treatment. We do not endorse any healthcare advice that contradicts a physician's guidance. Use of this site is subject to our Terms of Use, Privacy Policy, and Advertisement Policy. For more details, read our Full Disclaimer here.
NOTE: Join us in combating medical misinformation. If you encounter a questionable health, medical, or medical education claim, email us at factcheck@medicaldialogues.in for evaluation.