- Home
- Medical news & Guidelines
- Anesthesiology
- Cardiology and CTVS
- Critical Care
- Dentistry
- Dermatology
- Diabetes and Endocrinology
- ENT
- Gastroenterology
- Medicine
- Nephrology
- Neurology
- Obstretics-Gynaecology
- Oncology
- Ophthalmology
- Orthopaedics
- Pediatrics-Neonatology
- Psychiatry
- Pulmonology
- Radiology
- Surgery
- Urology
- Laboratory Medicine
- Diet
- Nursing
- Paramedical
- Physiotherapy
- Health news
- AYUSH
- State News
- Andaman and Nicobar Islands
- Andhra Pradesh
- Arunachal Pradesh
- Assam
- Bihar
- Chandigarh
- Chattisgarh
- Dadra and Nagar Haveli
- Daman and Diu
- Delhi
- Goa
- Gujarat
- Haryana
- Himachal Pradesh
- Jammu & Kashmir
- Jharkhand
- Karnataka
- Kerala
- Ladakh
- Lakshadweep
- Madhya Pradesh
- Maharashtra
- Manipur
- Meghalaya
- Mizoram
- Nagaland
- Odisha
- Puducherry
- Punjab
- Rajasthan
- Sikkim
- Tamil Nadu
- Telangana
- Tripura
- Uttar Pradesh
- Uttrakhand
- West Bengal
- Medical Education
- Industry
Automated machine learning may fast detect visual field loss patterns in glaucoma
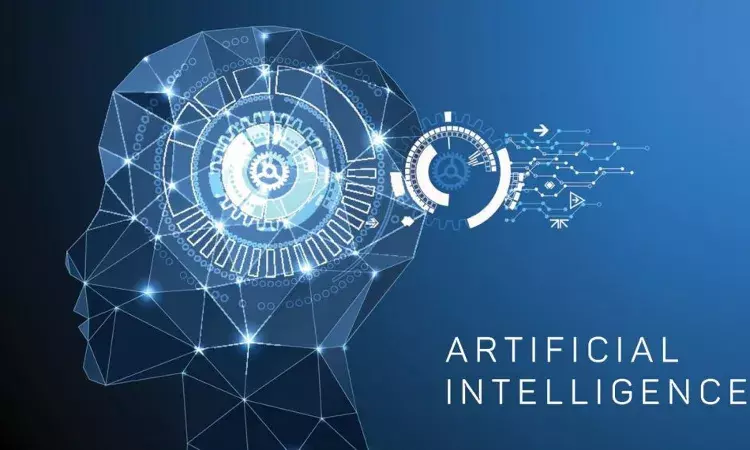
In a new study conducted by Siamak Yousefi and colleagues, it was found that an automated machine learning method can detect patterns of visual field (VF) loss and provide objective, reproducible terminology for describing early indicators of visual abnormalities and rapid progression in glaucoma patients. The findings of this study were published in Ophthalmology.
This was a cross-sectional and longitudinal study that followed 2231 aberrant VFs from 205 eyes of 176 OHTS individuals for almost 16 years. An unsupervised deep archetypal analysis method and an OHTS certified VF reader were used to discover common patterns of VF loss. Machine-identified glaucoma damage patterns were compared to those previously described (expert-identified) in the OHTS in 2003. The longitudinal VFs of each eye were used to identify VF loss patterns that were highly related to rapid glaucoma progression. The key findings of this study were machine-expert correlation and the types of patterns of VF loss linked with rapid growth.
The key findings of this study were as follows:
1. The average VF mean deviation (MD) at glaucoma conversion was -2.7 dB (SD = 2.4 dB), while the mean MD of the eyes at the previous visit was -5.2 dB (SD = 5.5 dB).
2. Fifty of the 205 eyes exhibited an MD rate of -1 dB/year or more and were classified as quick progressors.
3. Eighteen machine-identified VF loss patterns were compared to expert-identified patterns, with 13 VF loss patterns being identical.
4. The most common expert-identified patterns were temporal wedge, partial arcuate, nasal step, and paracentral VF flaws, while the most common machine-identified patterns were temporal wedge, partial arcuate, nasal step, and paracentral VF defects.
5. After adjusting for gender, age, and starting MD, one of the machine-identified patterns of VF loss indicated future rapid VF advancement.
In conclusion, the creation of a machine-learning artificial intelligence system would really be extremely beneficial in clinical care by objectively identifying VF abnormalities related with quicker glaucoma progression.
Reference:
Yousefi, S., Pasquale, L. R., Boland, M. V., & Johnson, C. A. (2022). Machine-identified Patterns of Visual Field Loss and An Association with Rapid Progression in the Ocular Hypertension Treatment Study. In Ophthalmology. Elsevier BV. https://doi.org/10.1016/j.ophtha.2022.07.001
Neuroscience Masters graduate
Jacinthlyn Sylvia, a Neuroscience Master's graduate from Chennai has worked extensively in deciphering the neurobiology of cognition and motor control in aging. She also has spread-out exposure to Neurosurgery from her Bachelor’s. She is currently involved in active Neuro-Oncology research. She is an upcoming neuroscientist with a fiery passion for writing. Her news cover at Medical Dialogues feature recent discoveries and updates from the healthcare and biomedical research fields. She can be reached at editorial@medicaldialogues.in
Dr Kamal Kant Kohli-MBBS, DTCD- a chest specialist with more than 30 years of practice and a flair for writing clinical articles, Dr Kamal Kant Kohli joined Medical Dialogues as a Chief Editor of Medical News. Besides writing articles, as an editor, he proofreads and verifies all the medical content published on Medical Dialogues including those coming from journals, studies,medical conferences,guidelines etc. Email: editorial@medicaldialogues.in. Contact no. 011-43720751