- Home
- Medical news & Guidelines
- Anesthesiology
- Cardiology and CTVS
- Critical Care
- Dentistry
- Dermatology
- Diabetes and Endocrinology
- ENT
- Gastroenterology
- Medicine
- Nephrology
- Neurology
- Obstretics-Gynaecology
- Oncology
- Ophthalmology
- Orthopaedics
- Pediatrics-Neonatology
- Psychiatry
- Pulmonology
- Radiology
- Surgery
- Urology
- Laboratory Medicine
- Diet
- Nursing
- Paramedical
- Physiotherapy
- Health news
- Fact Check
- Bone Health Fact Check
- Brain Health Fact Check
- Cancer Related Fact Check
- Child Care Fact Check
- Dental and oral health fact check
- Diabetes and metabolic health fact check
- Diet and Nutrition Fact Check
- Eye and ENT Care Fact Check
- Fitness fact check
- Gut health fact check
- Heart health fact check
- Kidney health fact check
- Medical education fact check
- Men's health fact check
- Respiratory fact check
- Skin and hair care fact check
- Vaccine and Immunization fact check
- Women's health fact check
- AYUSH
- State News
- Andaman and Nicobar Islands
- Andhra Pradesh
- Arunachal Pradesh
- Assam
- Bihar
- Chandigarh
- Chattisgarh
- Dadra and Nagar Haveli
- Daman and Diu
- Delhi
- Goa
- Gujarat
- Haryana
- Himachal Pradesh
- Jammu & Kashmir
- Jharkhand
- Karnataka
- Kerala
- Ladakh
- Lakshadweep
- Madhya Pradesh
- Maharashtra
- Manipur
- Meghalaya
- Mizoram
- Nagaland
- Odisha
- Puducherry
- Punjab
- Rajasthan
- Sikkim
- Tamil Nadu
- Telangana
- Tripura
- Uttar Pradesh
- Uttrakhand
- West Bengal
- Medical Education
- Industry
AI can detect lung cancers unnoticed in chest radiography: Study
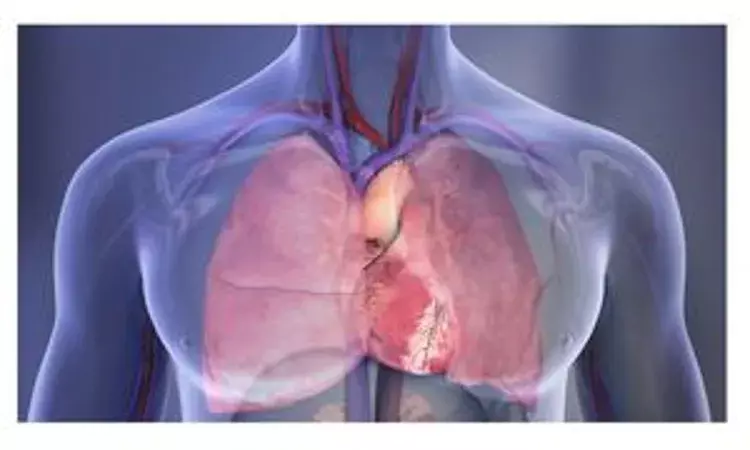
South Korea: A deep learning–based nodule detection algorithm can detect lung cancers that were initially not reported on chest radiographs, finds a recent study in the journal Radiology: Cardiothoracic Imaging. Additionally, the AI algorithm when used as a second reader can significantly reduce reading errors.
Ju Gang Nam, Seoul National University Hospital, South Korea, and colleagues evaluated the performance of a deep learning–based algorithm in detecting lung cancers not reported on posteroanterior chest radiographs during routine practice.
The retrospective test dataset included 168 posteroanterior chest radiographs acquired between March 2017 and December 2018 (168 patients) with 187 lung cancers undetected during initial clinical evaluation, and 50 normal chest radiographs. CT served as the reference standard for ground truth.
Four thoracic radiologists independently reevaluated the chest radiographs for lung nodules both without and with the aid of the algorithm. The performances of the algorithm and the radiologists were evaluated and compared on a per–chest radiograph basis and a per-lesion basis, according to the area under the receiver operating characteristic curve (AUROC) and area under the jackknife free-response ROC curve (AUFROC).
Key findings of the study include:
- The algorithm showed excellent diagnostic performances both in terms of per-chest radiograph classification (AUROC, 0.899) and per-lesion localization (AUFROC, 0.744); both of these values were significantly higher than those of the radiologists (AUROC, 0.634–0.663; AUFROC, 0.619–0.651).
- The algorithm also demonstrated higher sensitivity (69.6% vs 47.0% ) and specificity (94.0% vs 78.0%).
- When assisted by the algorithm, the radiologists' AUROC (0.634–0.663 vs 0.685–0.724) and pooled AUFROC (0.636 vs 0.688) substantially improved.
- The false-positive rate of the algorithm, that is, the total number of false-positive nodules divided by the total number of chest radiographs, was similar to that of pooled radiologists (21.1% vs 19.0%).
"A deep learning–based nodule detection algorithm showed excellent detection performance of lung cancers that were not reported on chest radiographs during routine practice and significantly reduced reading errors when used as a second reader," concluded the authors.
The study, "Undetected Lung Cancer at Posteroanterior Chest Radiography: Potential Role of a Deep Learning–based Detection Algorithm," is published in the journal Radiology: Cardiothoracic Imaging.
DOI: https://pubs.rsna.org/doi/full/10.1148/ryct.2020190222
Dr Kamal Kant Kohli-MBBS, DTCD- a chest specialist with more than 30 years of practice and a flair for writing clinical articles, Dr Kamal Kant Kohli joined Medical Dialogues as a Chief Editor of Medical News. Besides writing articles, as an editor, he proofreads and verifies all the medical content published on Medical Dialogues including those coming from journals, studies,medical conferences,guidelines etc. Email: drkohli@medicaldialogues.in. Contact no. 011-43720751